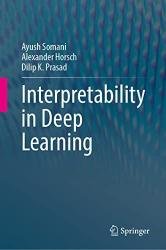
Автор: Ayush Somani, Alexander Horsch, Dilip K. Prasad
Издательство: Springer
Год: 2023
Страниц: 483
Язык: английский
Формат: pdf (true)
Размер: 12.1 MB
This book is a comprehensive curation, exposition and illustrative discussion of recent research tools for interpretability of Deep Learning models, with a focus on neural network architectures. In addition, it includes several case studies from application-oriented articles in the fields of Computer Vision, optics and Machine Learning related topic. The book can be used as a monograph on interpretability in Deep Learning covering the most recent topics as well as a textbook for graduate students. Scientists with research, development and application responsibilities benefit from its systematic exposition.