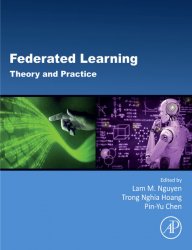
Автор: Lam M. Nguyen, Trong Nghia Hoang, Pin-Yu Chen
Издательство: Academic Press/Elsevier
Год: 2024
Страниц: 434
Язык: английский
Формат: epub
Размер: 23.4 MB
Federated Learning: Theory and Practice provides a holistic treatment to Federated Learning as a distributed learning system with various forms of decentralized data and features. Part I of the book begins with a broad overview of optimization fundamentals and modeling challenges, covering various aspects of communication efficiency, theoretical convergence, and security. Part II features emerging challenges stemming from many socially driven concerns of Federated Learning as a future public Machine Learning service. Part III concludes the book with a wide array of industrial applications of Federated Learning, as well as ethical considerations, showcasing its immense potential for driving innovation while safeguarding sensitive data. Federated Learning: Theory and Practice provides a comprehensive and accessible introduction to Federated Learning which is suitable for researchers and students in academia, and industrial practitioners who seek to leverage the latest advance in Machine Learning for their entrepreneurial endeavors. In our era of Big Data and the rapid advancement of Artificial Intelligence (AI), innovation and progress are often driven by harnessing the power of vast amounts of data. Yet, privacy concerns and data protection regulations have placed limitations on traditional centralized approaches to Machine Learning: While data are abundant, they often exist in small and isolated silos. The need for collaborative and privacy-preserving approaches to Machine Learning has therefore become more crucial than ever. In this context, Federated Learning (FL) has emerged as the de facto framework for distributed Machine Learning (ML) that preserves the privacy of data, especially in the proliferation of mobile and edge devices with their increasing capacity for storage and computation.