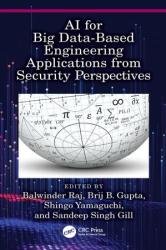
Автор: Balwinder Raj, Brij B. Gupta, Shingo Yamaguchi
Издательство: CRC Press
Год: 2023
Страниц: 261
Язык: английский
Формат: pdf (true)
Размер: 19.9 MB
Artificial Intelligence (AI), Machine Learning, and advanced electronic circuits involve learning from every data input and using those inputs to generate new rules for future business analytics. AI and Machine Learning are now giving us new opportunities to use Big Data that we already had, as well as unleash a whole lot of new use cases with new data types. With the increasing use of AI dealing with highly sensitive information such as healthcare, adequate security measures are required to securely store and transmit this information. This book provides a broader coverage of the basic aspects of advanced circuits design and applications.
AI for Big Data-Based Engineering Applications from Security Perspectives is an integrated source that aims at understanding the basic concepts associated with the security of advanced circuits. The content includes theoretical frameworks and recent empirical findings in the field to understand the associated principles, key challenges, and recent real-time applications of advanced circuits, AI, and Big Data security. It illustrates the notions, models, and terminologies that are widely used in the area of Very Large Scale Integration (VLSI) circuits, security, identifies the existing security issues in the field, and evaluates the underlying factors that influence system security. This work emphasizes the idea of understanding the motivation behind advanced circuit design to establish the AI interface and to mitigate security attacks in a better way for Big Data. This book also outlines exciting areas of future research where already existing methodologies can be implemented.
Th Chapter "Emerging Nonvolatile Memories for AI Applications" presents various types of emerging nonvolatile memories like FeFET-based memory, MRAM, STTRAM nanowire-based memory, etc. Nonvolatile memory devices have moved toward nanoscale size as a result of technological advancements and rising high-density demand. New products are urgently needed to overcome the present flash memory devices’ high programming voltage and current leakage issues. Carbon nanotube and nanowire have now been determined for different nonvolatile memory applications to be among the most significant nanomaterials, having exceptional mechanical and electrical characteristics. Comparisons of different nanowires on the basis of parameters such as diameter, length, mobility, and resistivity have been presented in this chapter. Furthermore, a comparative analysis of emerging nonvolatile memories (eNVM) devices has been carried out based on key performance metrics.
Power dissipation is the key factor that is considered while designing low-power circuits. Complementary metal oxide semiconductor (CMOS) technology faces major challenges in nanoscaled circuits, where power dissipation increases by a large amount. Quantum Dot Cellular Automata (QCA) is a prominent nanotechnology for the evolution of nanostructure-based circuits. In QCA nanotechnology, large amounts of power dissipation are mitigated by the concept of reversible computing. Reversible gates provide one-to-one mapping, therefore dissipating much less power. Different reversible gates in QCA nanotechnology have different structures and characteristics. This chapter compares various notable reversible gates and their results, which are investigated at logical and cell levels. The reversible gate designs are different in terms of the number of QCA cells, occupied area, and time delays. Various comparisons are performed for the cell count, occupied area, and the applied number of clock zones. Different reversible gates are presented and compared for the use in complex designs. Reversible gates having a smaller number of garbage outputs are preferred. The reversible gates are simulated using the QCA designer.
This material is suitable for students, researchers, and professionals with research interest in AI for Big Data–based engineering applications, faculty members across universities, and software developers.
Скачать AI for Big Data-Based Engineering Applications from Security Perspectives
