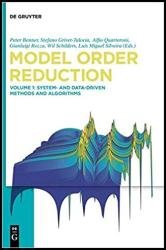
Автор: Peter Benner, Stefano Grivet-Talocia, Alfio Quarteroni
Издательство: De Gruyter
Год: 2021
Страниц: 388
Язык: английский
Формат: pdf (true), epub
Размер: 38.1 MB
An increasing complexity of models used to predict real-world systems leads to the need for algorithms to replace complex models with far simpler ones, while preserving the accuracy of the predictions. This three-volume handbook covers methods as well as applications. This first volume focuses on real-time control theory, data assimilation, real-time visualization, high-dimensional state spaces and interaction of different reduction techniques.
This first volume starts with an introductory chapter to Model Order Reduction (MOR) in general as a very broad field of research, encompassing multiple techniques with applications in a wide variety of fields. This chapter serves two main purposes. On the one hand, it provides an introduction to the handbook project itself, helping the reader navigate through the three volumes, explaining their organization, providing pointers into the various chapters where specific methods are presented or where particular applications are further explored. Additionally, the first chapter also serves as a conduit to introduce concepts and notation used throughout the various chapters and volumes, in an attempt to support, simplify and enrich the reader’s experience when probing the information provided in the three volumes of “Model Order Reduction”.
After this initial, introductory chapter, all chapters of this first volume mostly focus on the concept of MOR applied in a system-theoretical context. The common principle among methods and algorithms in this setting is the basic assumption that there is an underlying system description whose behavior can be determined from the knowledge of the dynamics of a set of state variables. Specific developments both in theory and applications, including deployment in commercial CAD tools, took place over the years in specific settings and disciplines, sometimes using different language and notation. However, all such methods share a common framework, which we attempted to capture in this book.
The ever-increasing demand for realistic simulations of complex products and processes places a heavy burden on the shoulders of mathematicians and, more generally, researchers and engineers working in the area of computational science and engineering (CSE). Realistic simulations imply that the errors of the virtual models should be small, and that different aspects of the product or process must be taken into account, resulting in complex coupled simulations. Often, there is a lot of superfluous detail in these simulations that is not needed to provide accurate results. With the current advances in new computer architectures, viz. the availability of many processors, one might be tempted to just use the abundant computational resources. However, this could lead to enormous energy consumption for the simulations, which should be avoided if possible. Besides, it could still lead to very lengthy and time-consuming simulations. Hence, it seems wise to use methods that can reduce the size of such huge problems, and which are able to get rid of the superfluous and unnecessary detail, still guaranteeing the accuracy of solutions.
An example is provided by the co-simulation of an electronic circuit with the interconnect structure that is mounted on top of the circuit to provide all desired connections. The metallic interconnect structure causes electromagnetic effects that may influence the behavior of the underlying circuit. There is no need, however, to solve the Maxwell equations for this complex three-dimensional structure in full detail, only the most dominant effects causing delays need to be included. This is where model order reduction (MOR) comes into play; MOR methods are able to extract the dominant behavior by reducing the size of the system to be solved.
Скачать Model Order Reduction: System- and Data-Driven Methods and Algorithms, Volume 1
