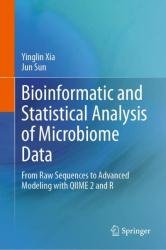
Автор: Yinglin Xia, Jun Sun
Издательство: Springer
Год: 2023
Страниц: 716
Язык: английский
Формат: pdf (true), epub
Размер: 33.7 MB
This unique book addresses the bioinformatic and statistical modelling and also the analysis of microbiome data using cutting-edge QIIME 2 and R software. It covers core analysis topics in both bioinformatics and statistics, which provides a complete workflow for microbiome data analysis: from raw sequencing reads to community analysis and statistical hypothesis testing. It includes real-world data from the authors’ research and from the public domain, and discusses the implementation of QIIME 2 and R for data analysis step-by-step. The data as well as QIIME 2 and R computer programs are publicly available, allowing readers to replicate the model development and data analysis presented in each chapter so that these new methods can be readily applied in their own research.
Bioinformatic and Statistical Analysis of Microbiome Data is an ideal book for advanced graduate students and researchers in the clinical, biomedical, agricultural, and environmental fields, as well as those studying bioinformatics, statistics, and Big Data analysis.
Statistical tools for performing microbiome data analysis are now available in different languages and environments across different platforms, either in web-based or programming-based approaches. Obviously, R system and environment play a critical role in developing statistical methods and models for analyzing microbiome data. QIIME 2 is a bioinformatic analysis tool via wrapping other sequencing platforms and also provides basic statistical analysis. Because of its comprehensive features and documentation supporting, QIIME 2 is one of the most popular bioinformatic tools in analysis of microbiome data. Thus, in this book, we leverage the capabilities of R and QIIME 2 for bioinformatic and statistical analysis of microbiome data.
Our book with 18 chapters is organized in this way: in the beginning two chapters, we specially provide overview and introduction of QIIME 2 and R in analysis of microbiome data, respectively. Chapters 3 to 6 present bioinformatic analysis of microbiome data and mainly through QIIME 2.
Chapter 7 introduces the original Operational Taxonomic Unit (OTU) methods in numerical taxonomy and Chapter 8 describes a movement of moving beyond OTU methods that has arisen in microbiome research field. Chapters 9 to 18 present biostatistical analysis of microbiome data and mainly through R and also QIIME 2.
Chapter 3 describes the basic data processing in QIIME 2. Chapter 4 introduces how to build feature table and feature data from raw sequencing reads. Chapter 5 introduces assigning taxonomy and building phylogenetic tree. Chapter 6 introduces taxonomic classification of the representative sequences and how to cluster Operational Taxonomic Units (OTUs). Chapter 7 comprehensively describes the development of OTU methods in numerical taxonomy, which provides a theoretical background of the clustering-based OTU methods that are used in bioinformatic analysis of microbiome data. Chapter 8 describes a movement that moves beyond OTU methods arisen in microbiome research field, which provides a comprehensive review on bioinformatic analysis of microbiome data. Chapters 9 and 10 provide two basic statistical analyses of microbiome dаta: Chap. 9 introduces alpha diversity metrics and visualization, Chap. 10 introduces beta diversity metrics and ordination. Chapters 11 to 18 present more advanced statistical methods and models in microbiome research.
Chapter 11 introduces nonparametric methods for multivariate analysis of variance in ecological and microbiome data and statistical testing of beta diversity in microbiome data. Chapter 12 discusses differential abundance analysis of microbiome data mainly through the metagenomeSeq package. Chapter 13 presents zero-inflated beta models for microbiome data. Chapter 14 introduces compositional data and specifically the newly developed models for compositional analysis of microbiome data. Chapter 15 introduces linear mixed-effects models and describes using them for analysis of longitudinal microbiome data. Chapter 16 describes generalized linear mixed models (GLMMs) including the brief history of generalized linear models and generalized nonlinear models, algorithms for fitting GLMMs, as well as statistical hypothesis testing and modeling in GLMMs. Chapter 17 specifically introduces the newly developed GLMMs for longitudinal microbiome data and adopting the GLMMs in other fields to analyze longitudinal microbiome data. Chapter 18 provides an overview of multivariate longitudinal microbiome data analysis and specifically introduces the newly developed non-parametric microbial interdependence test. The large P small N problem is also discussed in the last chapter of this book.
Contents:
Скачать Bioinformatic and Statistical Analysis of Microbiome dаta: From Raw Sequences to Advanced Modeling with QIIME 2 and R
