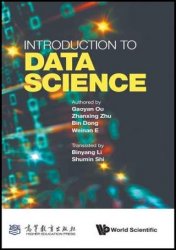
Автор: Gaoyan Ou, Zhanxing Zhu, Bin Dong
Издательство: World Scientific Publishing
Год: 2024
Страниц: 445
Язык: английский
Формат: pdf (true)
Размер: 32.9 MB
Data Science is an emerging discipline which emphasizes the cultivation of Big Data talents with interdisciplinary ability. The book systematically introduces the basic contents of Data Science, including data preprocessing and basic methods of data analysis, handling special problems (e.g. text analysis), Deep Learning, and distributed systems. In addition to systematically introducing the basic content of Data Science from a theoretical point of view, the book also provides a large number of data analysis practice cases.
Its purpose is to comprehensively introduce models and algorithms in Data Science from a technical point of view. This book systematically introduces the basic theoretical content of Data Science, including data preprocessing, basic methods of data analysis, processing of special problems (such as text analysis), Deep Learning, and distributed systems. In addition, this book provides a large number of case studies for data analysis application practice. Students can conduct practical training and interact with data on the iData-Course platform.
Artificial Intelligence (AI) has become a field with many practical applications and active research attracting wide attentions from both academy and industry communities. We expect to simulate humans to automatically handle different types of tasks through Artificial Intelligence systems, such as understanding natural language, speech, image, video, and auxiliary medical diagnosis, etc. Deep Learning, which has been developed in the past decade, provides a promising solution for these tasks. An important nature of Deep Learning is the ability to learn the complex features or representations of data from the process of building a hierarchical network.
In Spark, data analysts do their work on data processing by using two types of operations defined on RDD: transformations and actions. Compared with MapReduce, Spark’s main advantage is to improve the performance of data processing. For example, researchers used Hadoop and Spark to train logistic regression models using the same training set, and found that Spark is more than 100 times more efficient. The main reason is that data in Spark is shared through memory, while through disk in Hadoop. Spark is not a substitute for Hadoop. Hadoop and Spark are complementary. When data analysts perform data processing and analysis, they can choose either MapReduce or Spark. The main difference is that Spark performs better than MapReduce on some iterative and interactive tasks.
Contents:
Скачать Introduction to Data Science
