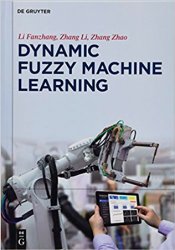
Автор: Li Fanzhang, Zhang Li, Zhang Zhao
Издательство: De Gruyter
Год: 2017
ISBN: 9783110518702
Формат: pdf
Страниц: 323
Размер: 10,5 mb
Язык: English
To meet the requirements of the readers of this book, the course is published here over a total of seven chapters.
In the first chapter, the dynamic fuzzy machine learning model is discussed. This chapter is divided into six sections. In the first section, we define the problem. In the second section, we introduce the dynamic fuzzy machine learning model. In the third section, we study some related work. The fourth section presents the algorithm of the dynamic fuzzy machine learning system and the related process control model. In the fifth section, we introduce the dynamic fuzzy relational learning algorithm. The sixth section summarizes the chapter.
The second chapter describes the dynamic fuzzy autonomous learning subspace learning algorithm. This chapter is divided into four sections. In the first section, we analyse the current state of autonomous learning. In the second section, we present an autonomous learning subspace theoretical system based on DFL. In the third section, we introduce the autonomic subspace learning algorithm based on DFL. In the fourth section, we summarize this chapter.
The third chapter is devoted to fuzzy decision tree learning. This chapter is divided into six sections. In the first section, we examine the current state of decision tree learning. In the second section, we study the dynamic fuzzy lattice decision tree method. In the third section, we discuss special attribute processing for dynamic fuzzy decision trees. In the fourth section, we study the pruning strategy for dynamic fuzzy decision trees. In the fifth section, we describe some applications of dynamic fuzzy decision trees. The sixth section summarizes this chapter.
In the fourth chapter, we consider dynamic concepts based on dynamic fuzzy sets. This chapter is divided into seven sections. In the first section, we analyse the relation between dynamic fuzzy sets (DFS) and concept learning. In the second section, we introduce the model of DF concept representation. In the third section, we model the DF concept learning space, and the fourth section describes the concept learning model based on the DF lattice. In the fifth section, we present a concept learning model based on dynamic fuzzy decision tree. In the sixth section, we discuss some applications of the dynamic concept based on dynamic fuzzy sets and analyse their performance. In the seventh section, we summarize this chapter.
The fifth chapter concentrates on semi-supervised multi-task learning based on dynamic fuzzy learning. This chapter is divided into six sections. The first section introduces the notion of multi-task learning. In the second section, we describe the semi-supervised multi-task learning model. In the third section, we introduce a semi-supervised multi-task learning model based on DFS. In the fourth section, we introduce a dynamic fuzzy semi-supervised multi-task matching algorithm. The fifth section extends this to a dynamic fuzzy semi-supervised multi-task adaptive learning algorithm. The sixth section summarizes this chapter.
In the sixth chapter, we study dynamic fuzzy hierarchy learning. This chapter is divided into seven sections. The first section introduces the idea of hierarchical learning. In the second section, we describe the design of an inductive logic program. The third section discusses dynamic fuzzy hierarchical relational learning (HRL). In the fourth section, we study dynamic fuzzy tree HRL, and the fifth section discusses dynamic fuzzy graph HRL. In the sixth section, we give some applications of dynamic concepts based on dynamic fuzzy sets and analyse their performance. The seventh section summarizes this chapter.
In Chapter 7, we consider a multi-agent learning model based on DFL. This chapter is divided into five sections. The first section introduces multi-agent learning. In the second section, we introduce the agent mental model based on DFL. In the third section, we introduce the single agent learning algorithm based on DFL. The fourth section extends this idea to a multi-agent learning model based on DFL. In the fifth section, we summarize this chapter. This book systematically introduces the relevant content of dynamic fuzzy learning. It can be used as a reference book for senior college students and graduate students as well as college teachers and scientific and technical personnel involved in computer science, artificial intelligence, machine learning, automation, mathematics, management science, cognitive science, financial management, and data analysis. The text can also be used as the basis for a lecture course on dynamic fuzzy learning.
