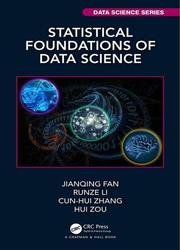
Автор: Jianqing Fan, Runze Li
Издательство: Chapman and Hall/CRC
Год: 2020
Страниц: 775
Язык: английский
Формат: pdf (true)
Размер: 34.3 MB
Statistical Foundations of Data Science gives a thorough introduction to commonly used statistical models, contemporary statistical Machine Learning techniques and algorithms, along with their mathematical insights and statistical theories. It aims to serve as a graduate-level textbook and a research monograph on high-dimensional statistics, sparsity and covariance learning, Machine Learning, and statistical inference. It includes ample exercises that involve both theoretical studies as well as empirical applications.
The book begins with an introduction to the stylized features of big data and their impacts on statistical analysis. It then introduces multiple linear regression and expands the techniques of model building via nonparametric regression and kernel tricks. It provides a comprehensive account on sparsity explorations and model selections for multiple regression, generalized linear models, quantile regression, robust regression, hazards regression, among others. High-dimensional inference is also thoroughly addressed and so is feature screening. The book also provides a comprehensive account on high-dimensional covariance estimation, learning latent factors and hidden structures, as well as their applications to statistical estimation, inference, prediction and machine learning problems. It also introduces thoroughly statistical machine learning theory and methods for classification, clustering, and prediction. These include CART, random forests, boosting, support vector machines, clustering algorithms, sparse PCA, and Deep Learning.
Deep Learning or deep neural networks has achieved tremendous success in recent years. In simple words, deep learning uses many compositions of linear transformations followed by a nonlinear gating to approximate high-dimensional functions. The family of such functions is very flexible so that they can approximate most of target functions very well. While neural networks have a long history, recent advances have greatly improved their performance in computer vision, natural language processing, machine translations, among others, where the information set x is given but highly complex such as images, texts and voices and the signal-to-noise ratio is high.
What makes Deep Learning so successful nowadays? The arrivals of Big Data allows us to reduce variance in the deep neural networks and modern computing architects and powers permit us to use deeper networks to better approximate high-dimensional functions and hence reduces the biases. In other words, Deep Learning is a great family of scalable nonparametric methods that achieve great bias and variance trade-off for high-dimensional function estimation when sample size is very large.
Скачать Statistical Foundations of Data Science
