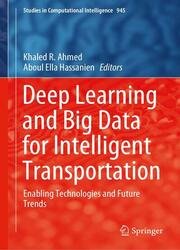
Автор: Khaled R. Ahmed, Aboul Ella Hassanien
Издательство: Springer
Год: 2021
Страниц: 264
Язык: английский
Формат: pdf (true), epub
Размер: 54.2 MB
This book contributes to the progress towards intelligent transportation. It emphasizes new data management and Machine Learning approaches such as Big Data, Deep Learning and reinforcement learning. Deep Learning and Big Data are very energetic and vital research topics of today’s technology. Road sensors, UAVs, GPS, CCTV and incident reports are sources of massive amount of data which are crucial to make serious traffic decisions. Herewith this substantial volume and velocity of data, it is challenging to build reliable prediction models based on Machine Learning methods and traditional relational database. Therefore, this book includes recent research works on Big Data, deep convolution networks and IoT-based smart solutions to limit the vehicle’s speed in a particular region, to support autonomous safe driving and to detect animals on roads for mitigating animal-vehicle accidents.
Chapter “Big Data Technologies with Computational Model Computing Using Hadoop with Scheduling Challenges” mainly focused on big data (BD) qualities and profoundly examined the complications raised by BD registering frameworks. The authors have clarified the estimation of big data mining in different areas. This chapter elaborates on the challenges incorporated with big data analytics (BDA) that are classified into data challenges, process challenges, and management challenges. Data challenges are a group of challenges related to data characteristics, such as volume, velocity, veracity, and visualization. Challenges that may be encountered while processing the data, such as data analysis, modeling, and data aggregation, are called process challenges. However, tackling privacy, security, operational cost, data governance, and data sharing are considered management challenges. This chapter gives a definite knowledge into the design, systems, and practices that are now continued in the big data process.
Chapter “Leveraging CNN Deep Learning Model for Smart Parking” leveraged the convolution neural network (CNN) deep learning model for smart parking. The authors trained the CNN algorithm to automate car parking to find empty and filled parking slots. The generated parking slot detection model’s efficiency is derived from the outcome of binary classification and recalling of the precision metrics.
This book serves broad readers including researchers, academicians, students and working professional in vehicles manufacturing, health and transportation departments and networking companies.
Contents:
I. Big Data and Autonomous Vehicles
Big Data Technologies with Computational Model Computing Using Hadoop with Scheduling Challenges
Big Data for Autonomous Vehicles
II. Deep Learning and Object Detection for Safe Driving
Analysis of Target Detection and Tracking for Intelligent Vision System
Enhanced End-to-End System for Autonomous Driving Using Deep Convolutional Networks
Deep Learning Technologies to Mitigate Deer-Vehicle Collisions
Night-to-Day Road Scene Translation Using Generative Adversarial Network with Structural Similarity Loss for Night Driving Safety
Safer-Driving: Application of Deep Transfer Learning to Build Intelligent Transportation Systems
Leveraging CNN Deep Learning Model for Smart Parking
Estimating Crowd Size for Public Place Surveillance Using Deep Learning
III. AI and IoT for Intelligent Transportation
IoT Based Regional Speed Restriction Using Smart Sign Boards
Synergy of Internet of Things with Cloud, Artificial Intelligence and Blockchain for Empowering Autonomous Vehicles
Combining Artificial Intelligence with Robotic Process Automation—An Intelligent Automation Approach
Скачать Deep Learning and Big Data for Intelligent Transportation: Enabling Technologies and Future Trends
