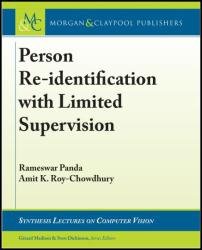
Автор: Rameswar Panda, Amit K Roy-Chowdhury
Издательство: Morgan & Claypool
Серия: Synthesis Lectures on Computer Vision
Год: 2021
Страниц: 100
Язык: английский
Формат: pdf (true)
Размер: 10.16 MB
Person re-identification is a very fast-growing field of research. This book considers a specific aspect of this problem, i.e., how to learn re-identification models with limited supervision. This is related to research in Machine Learning (ML) on developing unsupervised to weakly supervised approaches, given the huge effort required in supervision. While the book tries to provide an overview of the work in this area, it does not claim to be an exhaustive review of every possible paper in a very fast-developing area of research. Rather, it focuses on a set of specific research directions in learning re-identification models with limited supervision. In order to highlight some of the research trends, the book identifies some specific topics of interest. These are chosen so as to lead the reader to increasingly complex ideas.
First, we deal with the problem of how to minimize labeling effort in supervised approaches, followed by situations where the supervision is very limited, including one-shot supervision and unsupervised approaches. Thereafter, we consider the problem of dynamic camera networks where new camera(s) may be added or removed and show how learning-based approaches can be used in these settings, again with no to very limited supervision effort. There are many other directions that researchers should consider that are not described in detail in the book, and some of these are highlighted in the final chapter on future research directions.
In this book, we provide an overview of some of the literature in person re-identification, and then move on to focus on some specific problems in the context of person re-identification with limited supervision in multi-camera environments. We expect this to lead to interesting problems for researchers to consider in the future, beyond the conventional fully supervised setup that has been the framework for a lot of work in person re-identification.
Chapter 1 starts with an overview of the problems in person re-identification and the major research directions. We provide an overview of the prior works that align most closely with the limited supervision theme of this book. Chapter 2 demonstrates how global camera network constraints in the form of consistency can be utilized for improving the accuracy of camera pair-wise person re-identification models and also selecting a minimal subset of image pairs for labeling without compromising accuracy. Chapter 3 presents two methods that hold the potential for developing highly scalable systems for video person re-identification with limited supervision. In the one-shot setting where only one tracklet per identity is labeled, the objective is to utilize this small labeled set along with a larger unlabeled set of tracklets to obtain a re-identification model. Another setting is completely unsupervised without requiring any identity labels. The temporal consistency in the videos allows us to infer about matching objects across the cameras with higher confidence, even with limited to no supervision. Chapter 4 investigates person re-identification in dynamic camera networks. Specifically, we consider a novel problem that has received very little attention in the community but is critically important for many applications where a new camera is added to an existing group observing a set of targets. We propose two possible solutions for on-boarding new camera(s) dynamically to an existing network using transfer learning with limited additional supervision. Finally, Chapter 5 concludes the book by highlighting the major directions for future research.
Скачать Person Re-Identification with Limited Supervision
