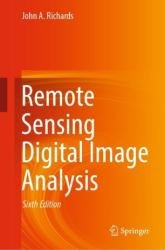
Автор: John A. Richards
Издательство: Springer
Год: 2022
Страниц: 576
Язык: английский
Формат: pdf (true)
Размер: 12.2 MB
Remote Sensing Digital Image Analysis provides a comprehensive treatment of the methods used for the processing and interpretation of remotely sensed image data. Over the past decade there have been continuing and significant developments in the algorithms used for the analysis of remote sensing imagery, even though many of the fundamentals have substantially remained the same. As with its predecessors this new edition again presents material that has retained value but also includes newer techniques, covered from the perspective of operational remote sensing.
Although the principal focus of the treatment is on digital image interpretation and the analytical techniques that make that possible, the material is located within the domain of remote sensing applications. That means project objectives are as important as finding the best-performing algorithm. Algorithms need to be incorporated into methodologies that can generate optimal results from a careful combination of procedures, and in which the steps of choosing reference material to support the process and for assessing accuracy, may be just as important as algorithm performance. While algorithm performance is a key objective in the Machine Learning (ML) remote sensing research community, it is project outcomes that drive the remote sensing applications specialist. That is a key emphasis of this book.
The classification techniques treated in Chap. 8 all require the availability of labelled training data with which the parameters of the respective class models are estimated. As a result, they are called supervised techniques because, in a sense, the analyst supervises an algorithm’s learning about those parameters. Sometimes labelled training data is not available and yet it would still be of interest to convert remote sensing image data into a thematic map of labels. Such an approach is called unsupervised classification since the analyst, in principle, takes no part in an algorithm’s learning process. Several methods are available for unsupervised learning. Perhaps the most common in remote sensing are based on the use of clustering algorithms, which seek to identify pixels in an image that are spectrally similar. That is one of the applications of clustering treated in the Chapter 9.
The book is designed as a teaching text for the senior undergraduate and postgraduate student, and as a fundamental treatment for those engaged in research using digital image analysis in remote sensing. The presentation level is for the mathematical non-specialist. Since the very great number of operational users of remote sensing come from the earth sciences communities, the text is pitched at a level commensurate with their background.
The chapters progress logically through means for the acquisition of remote sensing images, techniques by which they can be corrected, and methods for their interpretation. The prime focus is on applications of the methods, so that worked examples are included and a set of problems conclude each chapter.
Скачать Remote Sensing Digital Image Analysis, 6th Edition
