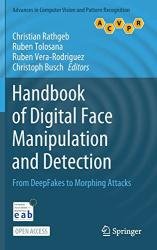
Автор: Christian Rathgeb, Ruben Tolosana
Издательство: Springer
Серия: Advances in Computer Vision and Pattern Recognition
Год: 2022
Страниц: 481
Язык: английский
Формат: pdf (true)
Размер: 13.5 MB
This book provides the first comprehensive collection of studies dealing with the hot topic of digital face manipulation such as DeepFakes, Face Morphing, or Reenactment. It combines the research fields of biometrics and media forensics including contributions from academia and industry. Appealing to a broad readership, introductory chapters provide a comprehensive overview of the topic, which address readers wishing to gain a brief overview of the state-of-the-art. Subsequent chapters, which delve deeper into various research challenges, are oriented towards advanced readers. Moreover, the book provides a good starting point for young researchers as well as a reference guide pointing at further literature. Hence, the primary readership is academic institutions and industry currently involved in digital face manipulation and detection. The book could easily be used as a recommended text for courses in image processing, Machine Learning, media forensics, biometrics, and the general security area.
In Part I of this handbook, the reader is provided with introductory overview chapters focusing on the topics of face image and video manipulations and detection (Chap. 1), the impact of different manipulations and alteration methods on face recognition systems (Chap. 2) and general multimedia forensics before the deep learning era (Chap. 3). These chapters serve as points of entry addressing readers wishing to gain a brief overview of the current state of the art.
Part II deals with the generation of manipulated face content and its security implications on face recognition, including DeepFakes (Chaps. 4 and 5), face morphing (Chap. 6), adversarial face images (Chap. 7) and audio-to-video face generation (Chap. 8). Subsequently, Part III elaborates on face manipulation detection techniques, containing dedicated chapters on different state-of-the-art detection methods for synthetically generated face images (Chap. 9), DeepFakes videos (Chaps. 10–14), morphed face images (Chaps. 15 and 16) and retouched face images (Chap. 17). Chapters in Part II and III delve deeper into the topics of digital face manipulation and detection and are oriented towards advanced readers.
Eventually, Part IV focuses on further topics including the use of face manipulation for privacy enhancement and the detection thereof (Chap. 18), practical challenges of face manipulation in remote scenarios (Chap. 19) as well as social and ethical issues (Chap. 20). Finally, in a concluding chapter authored by different contributors to this handbook, open research problems and future trends are summarised (Chap. 21).
In recent years there have been astonishing advances in AI-based synthetic media generation. Thanks to Deep Learning methods it is now possible to generate visual data with a high level of realism. This is especially true for human faces. Advanced Deep Learning tools allow one to easily change some specific attributes of a real face or even create brand new identities. Although this opens up a large number of new opportunities, just think of the entertainment industry, it also undermines the trustworthiness of media content and supports the spread of fake identities over the internet. In this context, there is a fundamental need to develop robust and automatic tools capable of distinguishing synthetic faces from real ones. The scientific community is making a huge research effort in this field, proposing several interesting approaches. However, a universal detector is yet to come. Fundamentally, the research in this field is like a cat and mouse game, with new detectors that are designed to deal with powerful synthetic face generators, while the latter keep improving to produce more and more realistic images. In the Chapter 9 we will present the most effective techniques proposed in the literature for the detection of synthetic faces. We will analyze their rationale, present real-world application scenarios , and compare different approaches in terms of accuracy and generalization ability.
Among the many applications of generative adversarial networks (GANs), image synthesis is one of the most investigated, and research in this field has shown a great potential. The visual appearance of the images generated by the latest GAN architectures is so realistic that it deceives even the experienced and attentive observer.
Скачать Handbook of Digital Face Manipulation and Detection: From DeepFakes to Morphing Attacks
