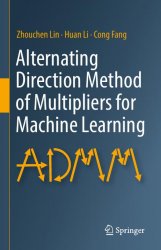
Автор: Zhouchen Lin, Huan Li, Cong Fang
Издательство: Springer
Год: 2022
Формат: PDF
Страниц: 274
Размер: 10 Mb
Язык: English
Machine learning heavily relies on optimization algorithms to solve its learning models. Constrained problems constitute a major type of optimization problem, and the alternating direction method of multipliers (ADMM) is a commonly used algorithm to solve constrained problems, especially linearly constrained ones. Written by experts in machine learning and optimization, this is the first book providing a state-of-the-art review on ADMM under various scenarios, including deterministic and convex optimization, nonconvex optimization, stochastic optimization, and distributed optimization. Offering a rich blend of ideas, theories and proofs, the book is up-to-date and self-contained. It is an excellent reference book for users who are seeking a relatively universal algorithm for constrained problems. Graduate students or researchers can read it to grasp the frontiers of ADMM in machine learning in a short period of time.
