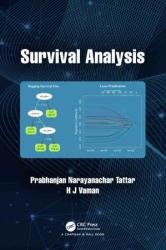
Автор: Prabhanjan Narayanachar Tattar, H.J. Vaman
Издательство: CRC Press
Год: 2023
Страниц: 373
Язык: английский
Формат: epub
Размер: 17,3 MB
Survival analysis generally deals with analysis of data arising from clinical trials. Censoring, truncation, and missing data create analytical challenges and the statistical methods and inference require novel and different approaches for analysis. Statistical properties, essentially asymptotic ones, of the estimators and tests are aptly handled in the counting process framework which is drawn from the larger arm of stochastic calculus. With explosion of data generation during the past two decades, survival data has also enlarged assuming a gigantic size. Most statistical methods developed before the millennium were based on a linear approach even in the face of complex nature of survival data. Nonparametric nonlinear methods are best envisaged in the Machine Learning school. This book attempts to cover all these aspects in a concise way.
Survival Analysis offers an integrated blend of statistical methods and Machine Learning (ML) useful in analysis of survival data. The purpose of the offering is to give an exposure to the Machine Learning trends for lifetime data analysis.
Why Machine Learning? That question might have occurred in the reader's mind before embarking on a journey of the vast body of machine learning techniques. A number of questions arise naturally. Should one enlist the techniques of this new paradigm? Does it generally provide more accurate results over the conventional statistical methods? Should the authors begin with statistical methods first and then write on Machine learning? In fact, we do not have to try too hard to justify the inclusion of Machine Learning (ML) methods in the book.
Features:
Classical survival analysis techniques for estimating statistical functional and hypotheses testing
Regression methods covering the popular Cox relative risk regression model, Aalen’s additive hazards model, etc.
Information criteria to facilitate model selection including Akaike, Bayes, and Focused
Penalized methods consisting of , , and elastic net
Survival trees and ensemble techniques of bagging, boosting, and random survival forests
A brief exposure of neural networks for survival data
R program illustration throughout the book
Скачать Survival Analysis
