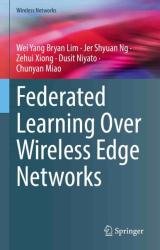
Автор: Wei Yang Bryan Lim, Jer Shyuan Ng, Zehui Xiong
Издательство: Springer
Год: 2022
Страниц: 175
Язык: английский
Формат: pdf
Размер: 10.1 MB
This book first presents a tutorial on Federated Learning (FL) and its role in enabling Edge Intelligence over wireless edge networks. This provides readers with a concise introduction to the challenges and state-of-the-art approaches towards implementing FL over the wireless edge network. Then, in consideration of resource heterogeneity at the network edge, the authors provide multifaceted solutions at the intersection of network economics, game theory, and Machine Learning towards improving the efficiency of resource allocation for FL over the wireless edge networks. A clear understanding of such issues and the presented theoretical studies will serve to guide practitioners and researchers in implementing resource-efficient FL systems and solving the open issues in FL respectively.
The confluence of edge computing and Artificial Intelligence (AI) has driven the rise of edge intelligence, which leverages the storage, communication, and computation capabilities of end devices and edge servers to empower AI implementation at scale closer to where data is generated. An enabling technology of edge intelligence is the privacy-preserving Machine Learning (ML) paradigm known as Federated Learning (FL). Amid the increasingly stringent privacy regulations, FL will enable the development of applications that have to be built using sensitive user data and will continue to revolutionize service delivery in finance, Internet of Things (IoT), healthcare, and transport industries, among others. However, the implementation of FL is envisioned to involve thousands of heterogeneous distributed end devices that differ in terms of communication and computation resources, as well as the levels of willingness to participate in the collaborative model training process. The potential node failures, device dropouts, and stragglers effect are key bottlenecks that impede the effective, sustainable, and scalable implementation of FL.
In Chap. 1, we will first present a tutorial and survey on FL and highlight its role in enabling edge intelligence. This tutorial and survey provide readers with a comprehensive introduction to the forefront challenges and state-of-the-art approaches towards implementing FL at the edge. In consideration of resource heterogeneity at the edge networks, we then provide multifaceted solutions formulated via the interdisciplinary interplay of concepts derived from network economics, optimization, game theory, and machine learning towards improving the efficiency of resource allocation for implementing FL at scale amid information asymmetry. In Chap. 2, we devise a multi-dimensional contract-matching approach for optimized resource allocation for federated sensing and learning amid multi-dimensional sources of heterogeneities. In Chap. 3, we propose a joint-auction coalition formation framework towards facilitating communication-efficient FL networks aided by unmanned aerial vehicles (UAVs). In Chap. 4, we propose a two-level evolutionary game theoretic and auction approach to allocate and price resources to facilitate efficient edge intelligence. In Chap. 5, we recap the key points and discuss the promising research directions for future works.
Скачать Federated Learning Over Wireless Edge Networks
