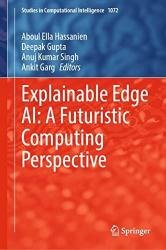
Автор: Aboul Ella Hassanien, Deepak Gupta, Anuj Kumar Singh
Издательство: Springer
Год: 2023
Страниц: 187
Язык: английский
Формат: pdf (true), epub
Размер: 19.4 MB
This book presents explainability in edge AI, an amalgamation of edge computing and AI. The issues of transparency, fairness, accountability, explainability, interpretability, data-fusion, and comprehensibility that are significant for edge AI are being addressed in this book through explainable models and techniques. The concept of explainable edge AI is new in front of the academic and research community, and consequently, it will undoubtedly explore multiple research dimensions. The book presents the concept of explainability in edge AI which is the amalgamation of edge computing and AI. In the futuristic computing scenario, the goal of explainable edge AI will be to execute the AI tasks and produce explainable results at the edge. First, this book explains the fundamental concepts of explainable artificial intelligence (XAI), then it describes the concept of explainable edge AI, and finally, it elaborates on the technicalities of explainability in edge AI. Owing to the quick transition in the current computing scenario and integration with the latest AI-based technologies, it is significant to facilitate people-centric computing through explainable edge AI. Explainable edge AI will facilitate enhanced prediction accuracy with the comprehensible decision and traceability of actions performed at the edge and have a significant impact on futuristic computing scenarios. This book is highly relevant to graduate/postgraduate students, academicians, researchers, engineers, professionals, and other personnel working in artificial intelligence, machine learning, and intelligent systems.
Explainable artificial intelligence (XAI) derives notions from different fields including cognitive sciences, philosophy, and psychology to yield a range of procedural methodologies that can produce explainable results for the end-users not having enough background on artificial intelligence. The main emphasis has been placed toward the progression of XAI that encompasses the human end-user in the cycle and, therefore, becomes human-centric. XAI-based intelligent systems will facilitate enhanced prediction accuracy with comprehensible decision and traceability of actions performed and have significant impact in the development of the society at large. In the futuristic computing scenario, the goal of explainable edge AI will be to execute the AI tasks and produce explainable results at the edge. The issues of transparency, fairness, accountability, explainability, interpretability, data fusion, and comprehensibility that are significant for the edge AI are being addressed here through explainable models and techniques. The book has been organized in ten chapters highlighting the different technological aspects of explainable edge AI.
Chapter 1 of the book provides a brief overview of explainable artificial intelligence (XAI) by covering almost every aspect of XAI. It covers the principles, techniques, current state of the art, benefits, and applications of XAI. It can be inferred from the chapter that XAI is a great development in AI due to its transparent nature. The chapter also addressed the challenges in XAI, as well as the field’s future potential. Chapter 2 of the book serves as a learning platform for academics and practitioners who are interested in essential parts of the new and quickly increasing field of XAI. The need of trust and transparency in AI and the principal application domain of XAI have been primarily discussed in this chapter. Chapter 3 epitomizes contemporary developments in explainable AI that describes explainability in machine learning, constituting a fiction definition of explainable machine learning that envelopes such prior conceptual propositions with a considerable focus on the audience for which the explainability is needed.
A wide and insightful view of XAI and its application in various fields has been presented in Chap. 4 of this book. This chapter also includes the future scope of this technology and the need for the growth of this type of technology. Chapter 5 presents recent challenges on edge AI and its numerous applications. This chapter provides a thorough analysis of edge computing-related AI techniques and tools, sometimes known as edge AI. The objective is to offer a hypothetical roadmap that may unite important players and enablers in order to the development of edge AI.
Chapter 6 highlights the usage of XAI in healthcare which is a critical application for the human community. ‘How XAI improves user trust in high-risk decisions’ has been elaborated in this chapter. Significant AI recommendations, such as surgical treatments or hospitalizations, requiring explanation from providers and patients have been discussed here. Chapter 7 focuses on describing the role of explainable edge AI to resolve real-time problems. Various issues in the applicability of explainable edge AI for real-time implementations have been highlighted in this chapter. Chapter 8 focuses on different data models of fusion, discusses a framework for AI and data fusion at the edge, and identifies potential challenges and possible solutions in this regard. While doing so, the chapter intends to cover the fundamentals of explainability in AI, the need to convert the black box system into a transparent one, and the associated opportunities for explainable artificial intelligence.
In Chap. 9 of this book, a novel technique in data fusion model with security and data optimization technique in edge computing has been presented. Here, the proposed data fusion is carried out using secure sequential discriminant autoencoder in which the improvement of data accuracy, as well as for the maximizing of edge cloud-based sensor networks lifespan. The fusion of edge cloud data has been carried out using discriminant autoencoder which is integrated with distributed edge cloud users, where the security of the network has been enhanced using secure sequential fuzzy-based trust model. The integration of edge computing framework with the sensor network that can aid in the data collection, dissemination, and decision making has been performed in Chap. 10 of the book. This chapter proposes a LSTM-based strategy for an edge computing-enabled WSN to determine the status of the node depending on the network parameters such as number of communications, number of packets transmitted, and initial energy of the nodes. The proposed protocol is implemented using TensorFlow and Keras libraries in Python language.
Скачать Explainable Edge AI: A Futuristic Computing Perspective
