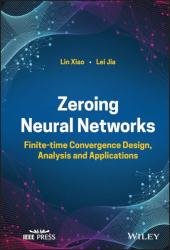
Автор: Lin Xiao, Lei Jia
Издательство: Wiley-IEEE
Год: 2023
Страниц: 435
Язык: английский
Формат: pdf (true), epub
Размер: 46.9 MB
Zeroing Neural Networks Describes the theoretical and practical aspects of finite-time ZNN methods for solving an array of computational problems. Zeroing Neural Networks (ZNN) have become essential tools for solving discretized sensor-driven time-varying matrix problems in engineering, control theory, and on-chip applications for robots. Building on the original ZNN model, finite-time zeroing neural networks (FTZNN) enable efficient, accurate, and predictive real-time computations. Setting up discretized FTZNN algorithms for different time-varying matrix problems requires distinct steps.
Computational problems usually play an important role in various fields. For example, matrix inversion problem is widely used in graph estimation, cloud computing, robotics, and image processing; matrix square root finding is widely applied in various scientific and engineering areas; linear matrix equations are widely used in control theory, optimization, signal processing, robotics, and multi‐agent systems; Lyapunov equation widely appears in many scientific and engineering fields, e.g. linear algebra, disturbance decoupling, and multi‐agent systems. For these computational problems, there are generally two kinds of solving methods: serial processing and parallel processing. In the previous studies, many numerical algorithms with the serial processing method are researched to solve the computational problems, such as Newton iteration and its variants, Meini iteration, singular value decomposition, and Greville recursive method. However, numerical algorithms have many limitations. For example, the computational complexity is usually proportional to the cube of the matrix dimension, which leads to the failure of solving large‐scale matrix problems in view of the limitations of numerical algorithms to solve constant matrix problems, let alone the efficiency for addressing time‐varying matrix problems. Actually, the time‐varying problems are more general in the real world, and thus their practical applications are more extensive. In recent years, due to the hardware‐implementation ability and parallel distributed nature of neural networks, they are developed vigorously by many researchers to solve computational problems, especially time‐varying problems.
Recurrent neural network (RNN) as a powerful computational tool has important applications, particularly in the fields of system identification, optimization, speech processing, and robotics, after the seminal work on Hopfield neural network. In algebraic computation, the RNN is divided into two classes: gradient neural network (GNN) and zeroing neural network (ZNN). The GNN method mainly adopts the norm of the error matrix, which is defined by the specific problems, as the performance indicator and designs a neural network evolving along the negative gradient‐descent direction to make the error norm decrease to zero with time.
Zeroing Neural Networks provides in-depth information on the finite-time convergence of ZNN models in solving computational problems. Divided into eight parts, this comprehensive resource covers modeling methods, theoretical analysis, computer simulations, nonlinear activation functions, and more. Each part focuses on a specific type of time-varying computational problem, such as the application of FTZNN to the Lyapunov equation, linear matrix equation, and matrix inversion. Throughout the book, tables explain the performance of different models, while numerous illustrative examples clarify the advantages of each FTZNN method. In addition, the book:
Describes how to design, analyze, and apply FTZNN models for solving computational problems
Presents multiple FTZNN models for solving time-varying computational problems
Details the noise-tolerance of FTZNN models to maximize the adaptability of FTZNN models to complex environments
Includes an introduction, problem description, design scheme, theoretical analysis, illustrative verification, application, and summary in every chapter
Zeroing Neural Networks: Finite-time Convergence Design, Analysis and Applications is an essential resource for scientists, researchers, academic lecturers, and postgraduates in the field, as well as a valuable reference for engineers and other practitioners working in neurocomputing and intelligent control.
Скачать Zeroing Neural Networks: Finite-time Convergence Design, Analysis and Applications
