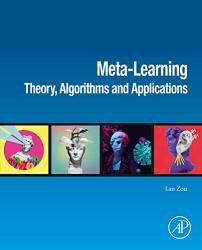
Автор: Lan Zou
Издательство: Academic Press/Elsevier
Год: 2023
Страниц: 404
Язык: английский
Формат: pdf (true), epub
Размер: 29.48 MB
Deep neural networks (DNNs) with their dense and complex algorithms provide real possibilities for Artificial General Intelligence (AGI). Meta-learning with DNNs brings AGI much closer: artificial agents solving intelligent tasks that human beings can achieve, even transcending what they can achieve. Meta-Learning: Theory, Algorithms and Applications shows how meta-learning in combination with DNNs advances towards AGI.
Meta-Learning: Theory, Algorithms and Applications explains the fundamentals of meta-learning by providing answers to these questions: What is meta-learning?; why do we need meta-learning?; how are self-improved meta-learning mechanisms heading for AGI ?; how can we use meta-learning in our approach to specific scenarios? The book presents the background of seven mainstream paradigms: meta-learning, few-shot learning, deep learning, transfer learning, machine learning, probabilistic modeling, and Bayesian inference. It then explains important state-of-the-art mechanisms and their variants for meta-learning, including memory-augmented neural networks, meta-networks, convolutional Siamese neural networks, matching networks, prototypical networks, relation networks, LSTM meta-learning, model-agnostic meta-learning, and the Reptile algorithm.
The book takes a deep dive into nearly 200 state-of-the-art meta-learning algorithms from top tier conferences (e.g. NeurIPS, ICML, CVPR, ACL, ICLR, KDD). It systematically investigates 39 categories of tasks from 11 real-world application fields: Computer Vision, Natural Language Processing, Meta-Reinforcement Learning, Healthcare, Finance and Economy, Construction Materials, Graphic Neural Networks, Program Synthesis, Smart City, Recommended Systems, and Climate Science. Each application field concludes by looking at future trends or by giving a summary of available resources.
Meta-Learning: Theory, Algorithms and Applications is a great resource to understand the principles of meta-learning and to learn state-of-the-art meta-learning algorithms, giving the student, researcher and industry professional the ability to apply meta-learning for various novel applications.
Machine Learning (ML) as a field is “concerned with the question of how to construct computer programs that automatically improve with experience.” A computer program is said to learn from experience E with respect to some class of tasks T and performance measure P, if its performance at tasks in T, as measured by P, improves with experience E. According to the characteristics of signal and feedback, Machine Learning approaches are commonly categorized into three groups: supervised learning, unsupervised learning, and reinforcement learning. Some literature includes semisupervised learning as a fourth approach. Supervised learning primarily relies on labeled training data in input-output pairs. Unsupervised learning draws inferences by extracting features from unlabeled training data. Reinforcement learning, in contrast, depends on rewards, states, and actions to manipulate the optimal policy. Semisupervised learning shares characteristics with supervised learning and unsupervised learning, consuming a mixture of extensive data without ground truth and limited annotated data. Some research associates these described paradigms with meta-learning.
A comprehensive overview of state-of-the-art meta-learning techniques and methods associated with deep neural networks together with a broad range of application areas
Coverage of nearly 200 state-of-the-art meta-learning algorithms, which are promoted by premier global AI conferences and journals, and 300 to 450 pieces of key research
Systematic and detailed exploration of the most crucial state-of-the-art meta-learning algorithm mechanisms: model-based, metric-based, and optimization-based
Provides solutions to the limitations of using deep learning and/or machine learning methods, particularly with small sample sizes and unlabeled data
Gives an understanding of how meta-learning acts as a stepping stone to Artificial General Intelligence in 39 categories of tasks from 11 real-world application fields
Скачать Meta-Learning: Theory, Algorithms and Applications
