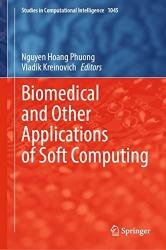
Автор: Nguyen Hoang Phuong, Vladik Kreinovich
Издательство: Springer
Серия: Studies in Computational Intelligence
Год: 2023
Страниц: 277
Язык: английский
Формат: pdf (true)
Размер: 10.2 MB
This book describes current and potential use of Artificial Intelligence (AI) and Computational Intelligence (CI) techniques in biomedicine and other application areas. Medical applications range from general diagnostics to processing of X-ray images to e-medicine-related privacy issues.
Medical community understandably prefers methods that have been successful other on other application areas, where possible mistakes are not that critical. This book describes many promising methods related to Deep Learning, fuzzy techniques, knowledge graphs, and Quantum Computing. It also describes the results of testing these new methods in communication networks, education, environmental studies, food industry, retail industry, transportation engineering, and many other areas.
In some cases, the existing Computational Intelligence techniques—often, after creative modifications—can be efficiently used in biomedical applications. However, biomedical problems are difficult. As a result, in many situations, the existing computational intelligence techniques are not sufficient to solve the corresponding problems. In such situations, we need to develop new techniques—and, ideally, first show their efficiency on other applications, to make sure that these techniques are indeed efficient. The fact that these techniques are efficient in so many different areas makes us hope that they will be useful in biomedical applications as well.
In this contribution, we establish a connection between a well-known mathematical structure called a manifold and a fuzzy partitioned space. We extend the apparatus of the theory of fuzzy (F-) transforms to 1-dimensional manifolds and thus make the first step in constructing data-driven F-transforms. With the overwhelming success of neural networks, we have witnessed a certain paradigm shift associated with the concept of data-driven versus model-driven. Generally speaking, this means that the data determines how it is processed. This manifests itself, for example, in a change in the focus of modeling from functions to features and from approximation to the Statistical Learning. It appears in the use of new proximity spaces, where a relaxed concept of closeness comes into play instead of traditional metric or normed vector spaces.
The goal of this work is to discover the generalization capabilities of topic models on new data and to quantify these information to ascertain, if topic models can contribute to Natural Language Processing (NLP) beyond their typical field of application as a purely descriptive tool. This paper therefore provides a blueprint for using the output of LDA Models and STMs for predictions, involving machine learning classifiers. We apply the concept by classifying Twitter social media posts into categories after pooling them based on the hashtag pooling algorithm of. The predictive power of the results is compared with the performance of state-of-the-art ANNs on the same classification task. Using topic model results as input for classifiers has many potential benefits: (i) the topic distribution itself becomes meaningful for the prediction, (ii) certain topics with high predictive power can be identified and different classifiers can be applied based on their accuracy, and (iii) statistical inference can be applied, for instance p-values or confidence intervals can be calculated. We show, that Machine Learning classifiers that are trained on a few topics, can match the capabilities and qualities of the classifications of ANNs.
This book helps practitioners and researchers to learn more about Computational Intelligence methods and their biomedical applications—and to further develop this important research direction.
Скачать Biomedical and Other Applications of Soft Computing
