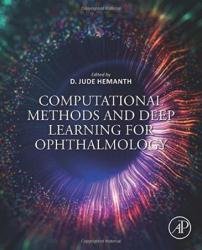
Автор: D. Jude Hemanth
Издательство: Academic Press/Elsevier
Год: 2023
Страниц: 354
Язык: английский
Формат: pdf
Размер: 17.9 MB
Computational Methods and Deep Learning for Ophthalmology presents readers with the concepts and methods needed to design and use advanced computer-aided diagnosis systems for ophthalmologic abnormalities in the human eye. Chapters cover computational approaches for diagnosis and assessment of a variety of ophthalmologic abnormalities. Computational approaches include topics such as Deep Convolutional Neural Networks, Generative Adversarial Networks, Auto Encoders, Recurrent Neural Networks, and modified/hybrid Artificial Neural Networks. Ophthalmological abnormalities covered include Glaucoma, Diabetic Retinopathy, Macular Degeneration, Retinal Vein Occlusions, eye lesions, cataracts, and optical nerve disorders.
This study explores the classification process using three CNNs, CifarNet, AlexNet, and GoogLeNet. The CNNs are examined for two different diseases Thoraco-abdominal lymph node and interstitial lung disease classification. This study shows that limited datasets can cause bottleneck problems. Therefore, large-scale annotated datasets can be beneficially classified using transfer learning models.
This work took a deep inspection on different requisites of Deep Learning in medical imaging. It suggested that deep convolutional neural networks (DCNNs) can auto-extract the mid and high-level features from the images. Increasing the number of iterations or epochs optimized the network parameters. When a medium-sized dataset is not available, then pretrained CNN is suggested and also the fine-tuning of the pretrained CNNs achieved better results. The challenges in medical imaging are need of a huge dataset, need of expensive medical expertise for high-quality annotation, and privacy issues in sharing the medical dataset.
This paper explained the processing operations to perform disease recognition using different approaches such as support vector machine (SVM), discrete cosine transform (DCT), hidden Markov model (HMM), and principal component analysis (PCA). The first step is the image acquisition followed by segmentation where the boundary of the iris is taken as circles, and they need not be cocentric. Then normalization of image is done to eliminate nonuniform illumination. Circular symmetric filter and grabber filter are used to extract features. Finally, a matching process is done by using encoding followed by hamming distance method.
Скачать Computational Methods and Deep Learning for Ophthalmology
