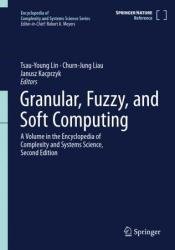
Автор: Tsau-Young Lin, Churn-Jung Liau, Janusz Kacprzyk
Издательство: Springer
Год: 2023
Страниц: 936
Язык: английский
Формат: pdf (true)
Размер: 26.2 MB
For this volume of ECSS, Second Edition, many entries have been updated to capture these new developments, together with new chapters on such topics as data clustering, outliers in data mining, qualitative fuzzy sets, and information flow analysis for security applications. Granulations can be seen as a natural and ancient methodology deeply rooted in the human mind. Many daily "things" are routinely granulated into sub "things": The topography of earth is granulated into hills, plateaus, etc., space and time are granulated into infinitesimal granules, and a circle is granulated into polygons of infinitesimal sides. Such granules led to the invention of calculus, topology and non-standard analysis. Formalization of general granulation was difficult but, as shown in this volume, great progress has been made in combing discrete and continuous mathematics under one roof for a broad range of applications in Data Science.
Soft computing was coined by Zadeh probably in the early 1980s to describe it as a consortium of the following areas: fuzzy logic (later augmented with rough sets), neural networks, and evolutionary computations. In fact, originally, probabilistic reasoning and Machine Learning were added, but this had not been followed as these areas concern different problems and use different tools and techniques. It should be noticed that these ingredients of soft computing have a common feature, namely, are meant to model and solve problems for which they do not presuppose to have exact models exemplified by complex models based on differential equations and strict optimization.
Though the term soft computing is still widely used, Computational Intelligence is getting more and more popular and widely employed, to a large extent due to the existence of the Computational Intelligence Society within the Institute of Electrical and Electronic Engineering (IEEE), the world’s largest engineering organization. There, the mission of Computational Intelligence is stated as to advance nature-inspired computational paradigms in science and engineering, while the field of interest is said to be the theory, design, application, and development of biologically and linguistically motivated computational paradigms emphasizing neural networks, connectionist systems, genetic algorithms, evolutionary programming, fuzzy systems, and hybrid intelligent systems, to be more specific neural networks, evolutionary computations of all kinds, fuzzy sets and fuzzy logic (with rough sets), ant and swarm type algorithms, “deeper” nature-inspired paradigms like the immunological paradigm, chaotic systems and computations, harmony search algorithms, probabilistic reasoning, etc. These topics expand those initially included in soft computing.
In recent years, hybrid neural networks such as fuzzy neural network, granular neural networks, and genetic neural networks have been investigated to solve complex application problems with high dimensionality. However, a single-stage hybrid neural network suffers from curse of dimensionality. A single-stage hybrid neural network suffers from curse of dimensionality because the number of parameters of the single-stage hybrid neural network is increasing exponentially with the increasing of the number of inputs. The hierarchical network structures based on multistage fuzzy reasoning have been used to solve the dimensionality problem.
Скачать Granular, Fuzzy, and Soft Computing
