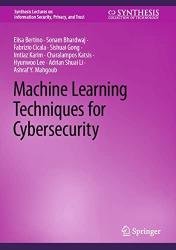
Автор: Elisa Bertino, Sonam Bhardwaj, Fabrizio Cicala, Sishuai Gong
Издательство: Springer
Год: 2023
Страниц: 169
Язык: английский
Формат: pdf (true), epub
Размер: 12.3 MB
This book explores Machine Learning (ML) defenses against the many cyberattacks that make our workplaces, schools, private residences, and critical infrastructures vulnerable as a consequence of the dramatic increase in botnets, data ransom, system and network denials of service, sabotage, and data theft attacks. The use of ML techniques for security tasks has been steadily increasing in research and also in practice over the last 10 years. Covering efforts to devise more effective defenses, the book explores security solutions that leverage Machine Learning (ML) techniques that have recently grown in feasibility thanks to significant advances in ML combined with big data collection and analysis capabilities. Since the use of ML entails understanding which techniques can be best used for specific tasks to ensure comprehensive security, the book provides an overview of the current state of the art of ML techniques for security and a detailed taxonomy of security tasks and corresponding ML techniques that can be used for each task.
It also covers challenges for the use of ML for security tasks and outlines research directions. While many recent papers have proposed approaches for specific tasks, such as software security analysis and anomaly detection, these approaches differ in many aspects, such as with respect to the types of features in the model and the dataset used for training the models. In a way that no other available work does, this book provides readers with a comprehensive view of the complex area of ML for security, explains its challenges, and highlights areas for future research. This book is relevant to graduate students in computer science and engineering as well as information systems studies, and will also be useful to researchers and practitioners who work in the area of ML techniques for security tasks.
Today, terms such as Artificial Intelligence (AI), Machine Learning (ML), and Deep Learning (DL) are widely used not only in the technical literature but also in the media, popular culture, advertising, and more. These terms are often used interchangeably. There are however differences among the respective areas that we outline in what follows.
Early AI approaches were mainly based on declarative knowledge provided by humans, for example, in terms of logical rules and ontologies. Such knowledge would then be used as input by inference mechanisms, often based on some formal logic. Today, AI encompasses a broad set of technology solutions that can learn on their own. A major problem of early AI approaches was the lack of scalability because of their reliance on human inputs. ML techniques, which started to be widely used in the1980s, address this problem by relying on data, instead of explicit human input. They apply statistical methodologies to identify patterns occurring in data. They improve their prediction tasks every time they acquire new data. A special category of ML techniques is represented by data mining (DM), which basically addressed the problem of identifying patterns on very large datasets.
DL techniques represent an important category of ML techniques that address the shortcoming of early ML techniques. DL essentially refers to algorithms that adapt, when exposed to different situations or data patterns. Vaguely inspired by biological neural networks, DL algorithms try to learn various characteristics from data and use them for decision-making/prediction on similar unseen data. DL techniques have gained interest because of the increased amounts of data available and their various algorithmic innovations as well as significant improvements in computing capabilities enabled by GPUs, which have made fast training and deployment of DL models possible.
Contents:
Скачать Machine Learning Techniques for Cybersecurity
