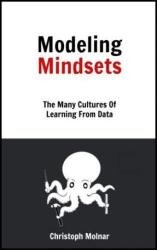
Автор: Christoph Molnar
Издательство: Mucbook
Год: 2022
Страниц: 113
Язык: английский
Формат: pdf (true), epub
Размер: 10.2 MB
In less than 100 pages, Modeling Mindsets elucidates the worldviews behind various statistical modeling and Machine Learning mindsets.
"Modeling Mindsets offers a compact but informative overview over the different approaches, assumptions and goals when working with data. I strongly recommend gifting this book to your present and future colleagues to get the most out of your collaboration."
– Sebastian Raschka, Machine learning & AI researcher
About The Book:
Most books on modeling dive straight into the math and methodologies, leaving readers struggling to grasp the underlying assumptions and limitations. Written in a clear and concise style, Modeling Mindsets introduces approaches such as Bayesian inference, supervised learning, causal inference, and more.
With this book, you'll gain a deeper understanding of different modeling techniques, empowering you to choose the right one for your problem.
Machine Learning (ML) is the branch of Artificial Intelligence (AI) that deals with improving at a given task through “experience,” which means learning from data. These tasks include clustering, planning, regression and classification, outlier detection, image generation, and text completion. In Machine Learning, there are not as many constraints on the model as in statistical modeling. Models are algorithms, not statistical hypotheses. Performance is valued over internal validity. For example, a Machine Learning may use random variables but doesn’t have to. The models can be neural networks, DBSCAN, Q-learning, decision trees, density estimators, statistical models and many more. Given this wide range of tasks and model classes: is machine learning a distinct mindset? To answer the question, let’s first look at specific mindsets within Machine Learning. Machine Learning is usually divided into supervised, unsupervised and reinforcement learning. Each of these subsets also represents a distinct modeling mindset: They involve a particular view of the world and of the relationship between the models and the world. The supervised learning mindset frames everything as a prediction problem and evaluates models by how well they perform on unseen data. In unsupervised learning, the goal is to find patterns in the data. The reinforcement learning mindset views the model as an actor in a dynamic environment guided by rewards. Deep Learning (DL) enables learning tasks end-to-end with neural networks.
"It has taken me many years of fumbling around with ML and statistics to achieve a fraction of the intuition in the book. Save yourself the time!"
– Robert Martin
Who This Book Is For:
Modeling Mindsets is designed for data scientists, statisticians, machine learners, and quantitative researchers who are already familiar with modeling and working with data.
To get the most out of this book:
You should already have experience with modeling and working with data.
You should feel comfortable with at least one of the mindsets in this book.
Don't read this book if:
You are completely new to working with data and models.
You cling to the mindset you already know and aren't open to other mindsets.
Embrace the full potential of Modeling Mindsets by challenging your assumptions and being open to diverse perspectives.
This compact guide is perfect for data-driven professionals looking to enhance their understanding of modeling approaches, assumptions, and goals when working with data. By delving into this book, you'll save years of trial and error, foster intellectual growth, and improve collaboration with your colleagues. Gain valuable insights into Bayesian inference, supervised learning, causal inference, and more, and elevate your data analysis skills by choosing the right modeling approach for your problem. Make the most of your data-modeling journey with "Modeling Mindsets."
Contents:
Скачать Modeling Mindsets: The Many Cultures Of Learning From Data
