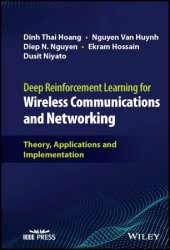
Автор: Dinh Thai Hoang, Nguyen Van Huynh, Diep N. Nguyen
Издательство: Wiley-IEEE Press
Год: 2023
Страниц: 288
Язык: английский
Формат: epub (true)
Размер: 12.5 MB
Comprehensive guide to Deep Reinforcement Learning (DRL) as applied to wireless communication systems.
Deep Reinforcement Learning empowered by deep neural networks (DNNs) has been developing as a promising solution to address high-dimensional and continuous control problems effectively. The integration of DRL into future wireless networks will revolutionize conventional model-based network optimization with model-free approaches and meet various application demands. By interacting with the environment, DRL provides an autonomous decision-making mechanism for the network entities to solve non-convex, complex, model-free problems, e.g. spectrum access, handover, scheduling, caching, data offloading, and resource allocation. This not only reduces communication overhead but also improves network security and reliability. Though DRL has shown great potential to address emerging issues in complex wireless networks, there are still domain-specific challenges that require further investigation. The challenges may include the design of proper DNN architectures to capture the characteristics of 5G network optimization problems, the state explosion in dense networks, multi-agent learning in dynamic networks, limited training data and exploration space in practical networks, the inaccessibility and high cost of network information, as well as the balance between information quality and learning performance.
Deep Reinforcement Learning for Wireless Communications and Networking presents an overview of the development of DRL while providing fundamental knowledge about theories, formulation, design, learning models, algorithms and implementation of DRL together with a particular case study to practice. The book also covers diverse applications of DRL to address various problems in wireless networks, such as caching, offloading, resource sharing, and security. The authors discuss open issues by introducing some advanced DRL approaches to address emerging issues in wireless communications and networking.
Covering new advanced models of DRL, e.g., deep dueling architecture and generative adversarial networks, as well as emerging problems considered in wireless networks, e.g., ambient backscatter communication, intelligent reflecting surfaces and edge intelligence, this is the first comprehensive book studying applications of DRL for wireless networks that presents the state-of-the-art research in architecture, protocol, and application design.
Deep Reinforcement Learning for Wireless Communications and Networking covers specific topics such as:
Deep reinforcement learning models, covering deep learning, deep reinforcement learning, and models of deep reinforcement learning
Physical layer applications covering signal detection, decoding, and beamforming, power and rate control, and physical-layer security
Medium access control (MAC) layer applications, covering resource allocation, channel access, and user/cell association
Network layer applications, covering traffic routing, network classification, and network slicing
With comprehensive coverage of an exciting and noteworthy new technology, Deep Reinforcement Learning for Wireless Communications and Networking is an essential learning resource for researchers and communications engineers, along with developers and entrepreneurs in autonomous systems, who wish to harness this technology in practical applications.
Contents:
Скачать Deep Reinforcement Learning for Wireless Communications and Networking: Theory, Applications and Implementation
