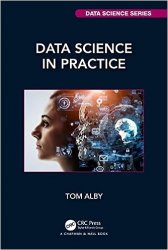
Автор: Tom Alby
Издательство: CRC Press
Год: 2024
Страниц: 318
Язык: английский
Формат: pdf (true)
Размер: 42.6 MB
Data Science in Practice is the ideal introduction to Data Science. With or without math skills, here, you get the all-round view that you need for your projects. This book describes how to properly question data, in order to unearth the treasure that data can be. You will get to know the relevant analysis methods, and will be introduced to the programming language R, which is ideally suited for data analysis. Associated tools like notebooks that make Data Science programming easily accessible are included in this introduction. Because technology alone is not enough, this book also deals with problems in project implementation, illuminates various fields of application, and does not forget to address ethical aspects. Data Science in Practice includes many examples, notes on errors, decision-making aids, and other practical tips. This book is ideal as a complementary text for university students, and is a useful learning tool for those moving into more data-related roles.
This English version has been updated to include the latest developments such as Quarto and ChatGPT. The rise of ChatGPT & Co raises voices as to whether Data Science is still necessary at all when Microsoft integrates AI into its Ofce product. Of course, Data Science will not disappear, because ChatGPT & Co can do many things really well, but special cases still have to be solved with the methods presented in this book.
Why Data Science with R? My passion for R started in the early 2000s when I was looking for a free software for data analysis and visualization. It was love at frst sight, even though I’ve written plenty of Python code since then, in addition to countless R programs. R is more in line with the way I think. I am sure that you will fall in love with R as well. In teaching practice, I have realized that the introduction to R is a lot faster than the introduction to Python, enabling me to get to the Data Science topics much faster. Data analysis is already hard enough for some, and then to get an introduction to programming ... In connection with R, most students do not even realize that they are just learning programming in addition to statistics. I usually need half a day for the introduction to R (see Chapter 4), the software library Tidyverse, which is popular in the data analysis feld, and notebooks (Chapter 5). Then, in the afternoon, students are introduced to data analysis (also Chapter 5). RStudio is also a wonderful integrated development environment that greatly simplifes data analysis. Such a tool, especially designed for data analysis, can be found in the Python world so far only in rudiments (for example in PyCharm). R is also much easier to install than Python, although kaggle.com and other platforms for both languages ofer a quick start via notebooks.
However, this does not mean that Python is less suitable for Data Science. It is not uncommon for R to be used to frst analyze the data and build initial models. This is exactly what we do in Chapters 6 to 9. For use in a productive environment, Python is often used, although this would also be possible with R. So it’s not an either/or but a both/and. And those who have learned R can learn Python all the more quickly.
Who Is This Book For?
I haven’t written this book for prospective computer scientists, but primarily for those who want to approach Data Science without having previously completed an advanced math course, even if they are not planning a career as a Data Scientist. Data Science will most likely be with us for some years to come, and therefore, it is immensely important that Data Scientists and stakeholders understand not only the possibilities but also the implications of Data Science. For those who want to go deeper into Data Science, this book ofers a compact introduction with examples and tips for further exercises and next steps on the way to becoming a professional Data Scientist. Chapters 10 to 12 ofer you additional content beyond the introduction to R and pure algorithms.
Key Features:
- Success factors and tools for all project phases
- Includes application examples for various subject areas
- Introduces many aspects of Data Science, from requirements analysis to data acquisition and visualization
Contents:
Скачать Data Science in Practice
