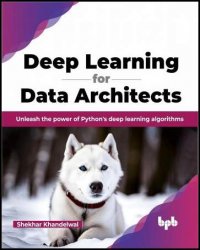
Автор: Shekhar Khandelwal
Издательство: BPB Publications
Год: 2024
Страниц: 544
Язык: английский
Формат: pdf, epub
Размер: 29.7 MB
A hands-on guide to building and deploying Deep Learning models with Python.
Key Features:
- Acquire the skills to perform exploratory data analysis, uncover insights, and preprocess data for Deep Learning tasks.
- Build and train various types of neural networks, including Convolutional Neural Networks (CNNs) and Recurrent Neural Networks (RNNs).
- Gain hands-on experience by working on practical projects and applying deep learning techniques to real-world problems.
Description:
“Deep Learning for Data Architects” is a comprehensive guide that bridges the gap between data architecture and Deep Learning. It provides a solid foundation in Python for data science and serves as a launchpad into the world of AI and Deep Learning.
The book begins by addressing the challenges of transforming raw data into actionable insights. It provides a practical understanding of data handling and covers the construction of neural network-based predictive models. The book then explores specialized networks such as convolutional neural networks (CNNs), recurrent neural networks (RNNs), and generative adversarial networks (GANs). The book delves into the theory and practical aspects of these networks and offers Python code implementations for each. The final chapter of the book introduces Transformers, a revolutionary model that has had a significant impact on natural language processing (NLP). This chapter provides you with a thorough understanding of how Transformers work and includes Python code implementations.
By the end of the book, you will be able to use Deep Learning to solve real-world problems.
What you will learn:
- Develop a comprehensive understanding of neural networks' key concepts and principles.
- Gain proficiency in Python as you code and implement major deep-learning algorithms from scratch.
- Build and implement predictive models using various neural networks
- Learn how to use Transformers for complex NLP tasks
- Explore techniques to enhance the performance of your deep learning models.
Chapter 1: Python for Data Science - serves as a solid foundation, providing an introduction to Python for data science. You will learn essential programming concepts, data structures, and libraries such as NumPy and Pandas. This chapter ensures that you are equipped with the necessary Python skills for the deep learning journey ahead.
Chapter 2: Real-World Challenges for Data Professionals in Converting Data Into Insights - dives into the challenges faced by data professionals when converting raw data into valuable insights. You will explore data cleaning, handling missing values, outlier detection, and feature engineering techniques. This chapter prepares you for the data preprocessing steps crucial for successful deep learning implementations.
Chapter 3: Build a Neural Network-Based Predictive Model - focuses on building predictive models using neural networks. You will learn about the architecture of a neural network, the role of activation functions, and techniques to handle classification and regression tasks. Through Python code implementations, you will gain hands-on experience in building and training neural networks.
Chapter 4: Convolutional Neural Networks - introduces CNNs, a powerful class of neural networks for image analysis tasks. You will understand the key components of CNNs, such as convolutional layers, pooling layers, and fully connected layers. The chapter provides Python code implementations to build CNN models for image classification tasks.
...
Chapter 10: Transformers - introduces Transformers, a revolutionary deep learning architecture that has gained prominence in natural language processing tasks. You will learn about the transformer architecture and its variants, such as BERT and GPT. Through Python code implementations, you will gain practical experience in applying transformers to text-related tasks.
Who this book is for:
This book is for anyone who is interested in a career in emerging technologies, such as Artificial Intelligence (AI), data analytics, Machine Learning, Deep Learning, and Data Science. It is a comprehensive guide that covers the fundamentals of these technologies, as well as the skills and knowledge that you need to succeed in this field.
Whether you are a seasoned data architect aiming to add deep learning to your arsenal, or a budding enthusiast stepping into the exciting intersection of these fields, this book is designed for you. As you turn the pages, you will find yourself not just learning, but evolving with the narrative of deep learning, setting the stage for a future-proof career in this dynamic domain.
Embrace this journey of learning and transformation, and let “Deep Learning for Data Architects” be your guide and companion in the exciting odyssey of AI and Data Science.
Chapter 1: Python for Data Science - serves as a solid foundation, providing an introduction to Python for data science. You will learn essential programming concepts, data struc
Скачать Deep Learning for Data Architects: Unleash the power of Python's deep learning algorithms
