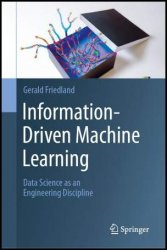
Автор: Gerald Friedland
Издательство: Springer
Год: 2024
Страниц: 281
Язык: английский
Формат: pdf (true), epub
Размер: 16.7 MB
This groundbreaking book transcends traditional Machine Learning approaches by introducing information measurement methodologies that revolutionize the field.
Stemming from a UC Berkeley seminar on experimental design for Machine Learning tasks, these techniques aim to overcome the 'black box' approach of Machine Learning by reducing conjectures such as magic numbers (hyper-parameters) or model-type bias. Information-based Machine Learning enables data quality measurements, a priori task complexity estimations, and reproducible design of Data Science experiments. The benefits include significant size reduction, increased explainability, and enhanced resilience of models, all contributing to advancing the discipline's robustness and credibility.
While bridging the gap between Machine Learning and disciplines such as physics, information theory, and computer engineering, this textbook maintains an accessible and comprehensive style, making complex topics digestible for a broad readership. Information-Driven Machine Learning explores the synergistic harmony among these disciplines to enhance our understanding of Data Science modeling. Instead of solely focusing on the "how," this text provides answers to the "why" questions that permeate the field, shedding light on the underlying principles of Machine Learning processes and their practical implications. By advocating for systematic methodologies grounded in fundamental principles, this book challenges industry practices that have often evolved from ideologic or profit-driven motivations. It addresses a range of topics, including Deep Learning, data drift, and MLOps, using fundamental principles such as entropy, capacity, and high dimensionality.
Deep Learning refers to training what is called a deep neural network (DNN). A DNN is an artificial neural network with multiple layers between the input and output layers. The most common type of DNN is a convolutional neural network (CNN), despite the fact that they deviate from the original idea of increasingly complex feature combination. CNNs are a specialized type of artificial neural networks that use convolution functions in place of threshold functions in at least one of their layers. They are specifically designed to process pixel or audio data (that is, noisy data) and are used in image/audio recognition and processing.
Machine Learning operations (MLOps) is a core function of Machine Learning engineering, focused on streamlining the process of taking machine learning models to production and then maintaining and monitoring them. The name is derived from DevOps (which stands for development operations) and is the process of testing and releasing software to production. What makes a good model is the same as what makes good software in general. Good software does not crash (exits without the user wanting it to exit), does not become unresponsive, and is able to warn and react well when the user provides inputs that seem to make no sense for the operations requested. In other words, the software (or the predictor) needs to be able to gracefully handle any random input. Just like any software, a model must be packaged together with all dependencies. Prediction must use the same preprocessing and tabularization as was done for training. This usually means the preprocessing needs to be packaged with the prediction model. If it is possible to exactly specify the kind of data that needs to be predicted correctly by a predictor, then a large part of the MLOps task can be fulfilled by a typical and frequently applied DevOps method: unit testing.
Скачать Information-Driven Machine Learning: Data Science as an Engineering Discipline
