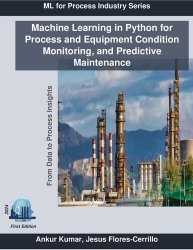
Автор: Ankur Kumar, Jesus Flores-Cerrillo
Издательство: Leanpub
Год: 2024-01-13
Страниц: 361
Язык: английский
Формат: pdf (true)
Размер: 18.0 MB
This book provides a guided tour of ML techniques utilized in process industry for plant health management. Step-by-step instructions, supported with industrial-scale process datasets, show how to develop ML-based solutions for equipment condition monitoring, plantwide monitoring, and predictive maintenance solutions.
This book is designed to help readers quickly gain a working knowledge of machine learning-based techniques that are widely employed for building equipment condition monitoring, plantwide monitoring, and predictive maintenance solutions in process industry. The book covers a broad spectrum of techniques ranging from univariate control charts to deep learning-based prediction of remaining useful life. Consequently, the readers can leverage the concepts learned to build advanced solutions for fault detection, fault diagnosis, and fault prognosis. The application focused approach of the book is reader friendly and easily digestible to the practicing and aspiring process engineers and data scientists. Upon completion, readers will be able to confidently navigate the Prognostics and Health Management literature and make judicious selection of modeling approaches suitable for their problems.
This book has been divided into seven parts. Part 1 lays down the basic foundations of ML-assisted process and equipment condition monitoring, and predictive maintenance. Part 2 provides in-detail presentation of classical ML techniques for univariate signal monitoring. Different types of control charts and time-series pattern matching methodologies are discussed. Part 3 is focused on the widely popular multivariate statistical process monitoring (MSPM) techniques. Emphasis is paid to both the fault detection and fault isolation/diagnosis aspects. Part 4 covers the process monitoring applications of classical machine learning techniques such as k-NN, isolation forests, support vector machines, etc. These techniques come in handy for processes that cannot be satisfactorily handled via MSPM techniques. Part 5 navigates the world of artificial neural networks (ANN) and studies the different ANN structures that are commonly employed for fault detection and diagnosis in process industry. Part 6 focusses on vibration-based monitoring of rotating machinery and Part 7 deals with prognostic techniques for predictive maintenance applications.
Jupyter notebooks and Spyder scripts with complete code implementations are available for download at GitHub.
Broadly, the book covers the following:
Exploratory analysis of process data
Best practices for process monitoring and predictive maintenance solutions
Univariate monitoring via control charts and time series data mining
Multivariate statistical process monitoring techniques (PCA, PLS, FDA, etc.)
Machine learning and deep learning techniques to handle dynamic, nonlinear, and multimodal processes
Fault detection and diagnosis of rotating machinery using vibration data
Remaining useful life predictions for predictive maintenance
Pre-requisites:
No prior experience with Machine Learning or Python is needed. Undergraduate-level knowledge of basic linear algebra and calculus is assumed.
Who should read this book:
The application-oriented approach in this book is meant to give a quick and comprehensive coverage of mainstream PM/PdM methodologies in a coherent, reader-friendly, and easy-to-understand manner. The following categories of readers will find the book useful:
1) Data scientists new to the field of process monitoring, equipment condition monitoring, and predictive maintenance
2) Regular users of commercial anomaly detection software (such as Aspen Mtell) looking to obtain a deeper understanding of the underlying concepts
3) Practicing process data scientists looking for guidance for developing process monitoring and predictive maintenance solutions
4) Process engineers or process engineering students making their entry into the world of Data Science
5) Industrial practitioners looking to build fault detection and diagnosis solutions for rotating machinery using vibration data
Скачать Machine Learning in Python for Process and Equipment Condition Monitoring, and Predictive Maintenance
