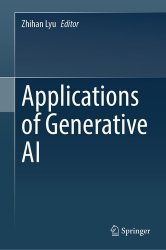
Автор: Zhihan Lyu
Издательство: Springer
Год: 2024
Страниц: 607
Язык: английский
Формат: pdf (true)
Размер: 21.7 MB
This book provides a comprehensive introduction to Generative AI in terms of basic concepts, core technologies, technical architecture, and application scenarios. Readers gain a deeper understanding of the emerging discipline of Generative AI. This book covers the latest cutting-edge application technologies of Generative AI in various fields. It provides relevant practitioners with ideas to solve problems and deepen their understanding of Generative AI. At the same time, it guides and helps Generative AI and related industries to deepen their understanding of the industry and enhance professional knowledge and skills. Starting from reality, this book lists many cases and analyzes theories in a popular image.
The Chapter 1 to bridge the gap between the theoretical potential of Generative AI (GAI) tools, such as Generative Pretrained Transformer (GPT), and their practical applications as supportive tools for scientific research. The chapter provides approaches and techniques for leveraging GAI to address research challenges and activities. It describes common research tasks and provides guidance on how to use GPT to solve them. To the best of our experience, at the current stage, the integration of researchers and GPT has the potential to yield better results than either could achieve alone. Furthermore, the increasing availability of GPT tools suggests that the synergy between the two will continue to improve research output quality and save time. The key to successful integration lies in the appropriate use of GPT that can be achieved by directing AI tools to solve tasks effectively and using prompt engineering techniques.
The GAI output is known as AI Generated Content (AIGC). Generative AI is a class of Artificial Intelligence (AI) models. At the time of writing these lines, GAI models’ cores are mainly based on Generative Adversarial Networks (GANs) and Transformer based models such as GPT-3, GPT-3.5 and GPT-4. GAI applications can be classified as open-source, private, and closed-source GAI. Open source is more relevant to specific enterprise needs and applications, whereas private and closed-source GAI are more general-oriented solutions to AI based on Large Language Models (LLM).
This chapter delves into the concepts of neural rendering and generative models, highlighting their importance in the fields of computer graphics, computer vision, and Artificial Intelligence. Neural rendering techniques utilize Deep Learning algorithms to generate realistic images, videos, or 3D models, while generative models learn the underlying data distribution to create novel content. The chapter explores various methodologies, such as Neural Radiance Fields, Variational Autoencoders, and Generative Adversarial Networks, among others. Applications of these techniques, such as photorealistic rendering, style transfer, and image synthesis, are discussed, along with the challenges and limitations associated with them. The chapter concludes with an outlook on the future prospects of neural rendering and generative models, emphasizing their potential to revolutionize digital content creation and consumption.
The book is useful for AI researchers and specifically for those working with the applications at hand (primarily medical imaging and construction/twinning industry). It covers a variety of cutting-edge technologies in Generative AI, which provides researchers with new research ideas.
Скачать Applications of Generative AI
