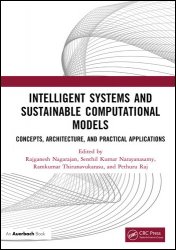
Автор: Rajganesh Nagarajan, Senthil Kumar Narayanasamy
Издательство: CRC Press
Год: 2024
Страниц: 429
Язык: английский
Формат: pdf (true)
Размер: 20.0 MB
The fields of intelligent systems and sustainability have been gaining momentum in the research community. They have drawn interest in such research fields as Computer Science, information technology, electrical engineering, and other associated engineering disciples. The promise of intelligent systems applied to sustainability is becoming a reality due to the recent advancements in the Internet of Things (IoT), Artificial Intelligence (AI), Big Data, Blockchain, Deep Learning, and Machine Learning. The emergence of intelligent systems has given rise to a wide range of techniques and algorithms using an ensemble approach to implement novel solutions for complex problems associated with sustainability.
Intelligent Systems and Sustainable Computational Models: Concepts, Architecture, and Practical Applications explores this ensemble approach towards building a sustainable future. It explores novel solutions for such pressing problems as smart healthcare ecosystems, energy efficient distributed computing, affordable renewable resources, mitigating financial risks, monitoring environmental degradation, and balancing climate conditions. The book helps researchers to apply intelligent systems to computational sustainability models to propose efficient methods, techniques, and tools.
Deep Learning and big data are two buzzwords that have taken the world of technology by storm. Deep Learning is a subset of machine learning that is a subset of Artificial Intelligence (AI), which trains neural networks to learn from large amounts of data. It is a self-learning process where the algorithms get better as they process more information. Deep Learning aims to create algorithms that can learn from data and forecast outcomes without explicit programming. Big Data refers to the massive amounts of information generated by businesses, governments, and individuals every day. This data can come from a variety of sources such as social media, Internet of Things (IoT) devices, customer interactions, etc. It is difficult to process and analyze this data using traditional methods due to its sheer volume, variety, and velocity.
Artificial Intelligence models have been widely adopted in all sectors. Still, their adoption has been tapering due to the transparency concerns required in mission-critical applications or applications relying on sensitive information. In most cases, Artificially Intelligent models remain a “black box” where even the programmers do not know how the final decision is attained. Various stakeholders of these systems, like programmers, users, and decision-makers, are puzzled at the prediction results and are concerned about the sensitive information fed to them. The systems must enforce transparency in decision-making to enhance stakeholders’ trust and guarantee faster adoption of Artificially Intelligent systems. The evolution of XAI has changed the prevailing trustworthiness concerns in adopting the AI models. XAI is decisive for an organization to build confidence and self-reliance when implementing AI models. More explainable AI helps organizations to adopt a liable approach to AI expansion.
XAI is a new technology encompassing various processes and approaches that allow human users and research practitioners to comprehend better and trust AI algorithms’ outcomes and experimental results. Though explainability and interpretability are often used interchangeably while conversing about AI, they differ technically. The later interpretable AI models interpret human decisions without supplementary information in considerable time. Also, human users can replicate the results with considerable data and time. Whereas the former, an explainable model, is intricate, requiring humans to lay extra effort into understanding and comprehending the decisions arrived. Sometimes it may require an analogy to help humans understand the model’s interpretations and the results. But, explainable models cannot be replicated even with infinite time and enormous data.
The book covers such areas as:
Intelligent and adaptive computing for sustainable energy, water, and transportation networks
Blockchain for decentralized systems for sustainable applications, systems, and infrastructure
IoT for sustainable critical infrastructure
Explainable AI (XAI) and decision-making models for computational sustainability
Sustainable development using edge computing, fog computing and cloud computing
Cognitive intelligent systems for e-learning
Artificial Intelligence and Machine Learning for large scale data
Green computing and cyber physical systems
Real-time applications in healthcare, agriculture, smart cities, and smart governance.
By examining how intelligent systems can build a sustainable society, the book presents systems solutions that can benefit researchers and professionals in such fields as information technology, health, energy, agricultural, manufacturing, and environmental protection.
Скачать Intelligent Systems and Sustainable Computational Models: Concepts, Architecture, and Practical Applications
