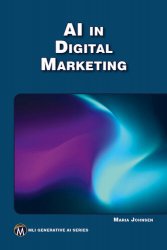
Автор: Maria Johnsen
Издательство: Mercury Learning and Information
Серия: MLI Generative AI Series
Год: 2024
Страниц: 539
Язык: английский
Формат: pdf, epub (true)
Размер: 10.1 MB
This book presents a comprehensive and innovative exploration of the role of Artificial Intelligence (AI) in the digital marketing arena. It begins with historical context and fundamental AI principles, and subsequently, details AI's applications across a spectrum of areas, including data analytics, content creation, customer targeting, Search Engine Optimization (SEO), Pay Per Click (PPC) advertising, Social Media Marketing (SMM), and Email Marketing. A distinctive feature lies in the author's extensive real-world experience, providing many useful business applications of AI. The book is designed for marketing professionals, business executives, educators, and students, and offers numerous examples and case studies.
Machine Learning and Deep Learning represent two of the most captivating and transformative domains within contemporary technology. Over the past decade, these fields have undergone rapid and profound development, exerting a profound influence across various industries and fundamentally altering our approach to intricate problem-solving. In this chapter, we will delve into the fundamental principles, methodologies, and practical applications that underpin these disciplines. Furthermore, we will scrutinize the salient disparities between them and explore their consequential real-world ramifications.
Machine Learning constitutes a subset of Artificial Intelligence (AI) that primarily concerns itself with endowing computers with the capacity to acquire knowledge from data. Rather than explicitly programming a computer to execute a task, Machine Learning models are trained to identify patterns within data and subsequently formulate predictions or decisions based on these discerned patterns.
Machine Learning algorithms constitute mathematical models and techniques designed to assimilate knowledge from data. Prominent Machine Learning algorithms include linear regression, decision trees, support vector machines, and k-nearest neighbors. Machine Learning models acquire knowledge through exposure to substantial volumes of labeled data. During the training process, the model adapts its internal parameters to minimize the disparities between its predictions and the actual data. Developing custom machine learning models is an option for businesses that want to create highly tailored customer profiles. Python libraries like Scikit-learn and TensorFlow are often used for this purpose.
The advent of the Internet and the proliferation of social media platforms have led to a substantial increase in the volume of data and text-based content available. In response to this dynamic informational landscape, natural language processing (NLP) has emerged as a transformative force within the domain of digital marketing. NLP, as a constituent subfield of Artificial Intelligence (AI), focuses on the intricate relationship between computational systems and human language. Through its capabilities, NLP empowers marketers to extract valuable insights from unstructured data, automate various processes, and enhance the quality of customer engagement. In this discourse, we will endeavor to elucidate the diverse applications of NLP within the domain of digital marketing and evaluate its implications for improving customer engagement. Natural language processing (NLP) is a subfield of artificial intelligence (AI) that assumes a central role in enabling computers to comprehend, interpret, and generate human language in a meaningful and valuable manner. NLP encompasses a wide range of tasks, including text analysis, sentiment analysis, machine translation, and the development of chatbots. Its algorithms are meticulously designed to analyze and process extensive volumes of textual data, thus facilitating the extraction of valuable insights and enabling real-time responses to customer inquiries. This chapter explores the applications of NLP in the field of digital marketing, assesses its impact on customer engagement, and outlines relevant challenges and considerations.
Several notable NLP tools are available to facilitate text analysis and related tasks:
Gensim: Renowned for its high speed and scalability, Gensim, a Python library, is primarily designed for topic modeling and excels in tasks such as the identification of textual similarities, document navigation, and text indexing.
SpaCy: An open-source Python NLP library known for its speed and comprehensive documentation, SpaCy handles sizable datasets and offers a wide array of pre-trained NLP models, making it particularly advantageous for text preparation in deep learning and information extraction.
IBM Watson: Comprising a versatile suite of AI-based services hosted within the IBM cloud infrastructure, IBM Watson is well-suited for tasks related to natural language understanding, encompassing functions such as keyword identification, emotion analysis, and categorization, making it a valuable asset in various industries.
The Natural Language Toolkit (NLTK): NLTK empowers users in the creation of Python programs tailored to human language data. It provides accessible interfaces to over 50 lexical and corpora resources, an array of text processing libraries, and a robust discussion forum, making it a widely adopted open-source platform.
Features:
• Underscores the significance of content marketing in the AI era, covering AI-driven content creation, content curation, and search engine optimization
• Examines social media marketing in detail, with valuable insights into social media listening, chatbots, using Google Ads, and influencer marketing
• Provides a comprehensive section on paid advertising and paid social media, revealing programmatic advertising, ad creative, and machine learning-driven ad campaign optimization
• Includes ethical considerations and legal aspects about privacy and data protection
Table of contents:
1: The Role of AI in Digital Marketing. 2: Understanding Artificial Intelligence. 3: Harnessing Data and Analytics in AI Digital Marketing. 4: Machine Learning and Deep Learning Foundation. 5: Natural Language Processing in Digital Marketing. 6: Personalization and Customer Segmentation with AI. 7: Predictive Analytics for Targeted Marketing. 8: Behavioral Analysis and Customer Profiling. 9: Content Creation with Artificial Intelligence. 10: Emotional Search Engine. 11: AI in Search Engines. 12: Enhancing Search Engine Optimization. 13: Social Media Listening and Sentiment Analysis. 14: Chatbots and Conversational AI. 15: Influencer Marketing in the Age of AI. 16: The Latest AI Trends in Digital Marketing. 17: Leveraging AI for Email Marketing Campaigns. 18: Marketing Automation and Workflow Optimization. 19: A/B Testing and Optimization with AI. 20: AI in Multichannel and Omnichannel Marketing. 21: Programmatic Advertising and Real-Time Bidding. 22: Creative Ad Design with AI. 23: Ad Campaign Optimization with Machine Learning. 24: AI-Powered Customer Service and Support. 25: Churn Prediction and Customer Retention Strategies. 26: Cross-Selling and Upselling with AI. 27: Privacy and Data Protection in AI Marketing. 28: Ethical AI Practices and Bias Mitigation. 29: Key Performance Indicators for AI Marketing. 30: ROI and Cost-Benefit Analysis. 31: Dynamic Factors Reshaping the Future of AI in Digital Marketing. Appendices. Glossary. About the Author. Index.
About the author:
Maria Johnsen is recognized as a leading figure in the digital realm and ranked by Onalytica among the top digital marketing influencers worldwide. She has penned 60 books on various subjects including digital marketing, filmmaking, artificial intelligence, and blockchain.
Скачать AI In Digital Marketing
