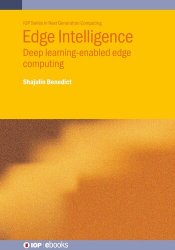
Автор: Shajulin Benedict
Издательство: IOP Publishing
Год: 2024
Страниц: 277
Язык: английский
Формат: pdf (true), epub
Размер: 31.7 MB
Edge Intelligence: Deep Learning-enabled edge computing is a book that targets researchers and practitioners who are interested in applying intelligence without compromising data privacy. The book reveals the existing edge-AI techniques and forecasts future edge-AI integration methods. The book delves into edge computing architectures after describing relevant basic technologies such as IoT, cloud computing, and other security-related architectures. The book starts with an explanation of all relevant basic technologies. It offers a smooth transition from the basics to insightful practical sessions for practitioners. The ideas of providing innovative ideas and applications in the later part of the book can enthuse researchers and developers to engage themselves in innovating newer products with the application of Edge Intelligence. Part of IOP Series in Next Generation Computing.
Edge intelligence is deployed in two broad ways: (i) machine learning-based intelligence; and (ii) deep learning-based intelligence. In this category, Machine Learning algorithms are deployed on edge nodes or devices to capture, learn, assess, and decide on key activities. These tasks need to be agile without any compromise on expected learning qualities. Edge devices need to automatically identify learning patterns, detect anomalies, and modify data behavior in real time by implementing machine learning algorithms and models at the edge. Traditional machine learning models such as random forests, support vector machines, Bayes theorem, etc, have limitations in processing data, i.e., the learning accuracy reduces with increasing input data. This is because Machine Learning algorithms are prone to overfitting and bias-related issues in many use cases.
Deep Learning-based edge intelligence: Deep Learning, in general, is a sub-field of Machine Learning that mimics the learning processes of humans. Our human brains learn different data based on several histories of information. This involves computationally powerful computers or computing devices to learn a large volume of data that arises from data-intensive applications, such as IoT-enabled applications. In recent years, the majority of real-world applications have included Deep Learning algorithms on edge nodes, considering the performance efficiency and learning accuracy with respect to the input regional data. The most widely applied Deep Learning algorithms are convolutional neural networks (CNNs), image segmentation algorithms, generative adversarial networks (GANs), reinforcement learning (RL), and transformers. The application of Deep Learning algorithms on edge-level nodes increases privacy and avoids latency while considering a large chunk of data in an automated fashion.
Transfer learning is widely utilized and implemented in several edge-enabled applications. Implementing similar transfer learning-based applications is easier using specialized frameworks such as TensorFlow. These frameworks have several in-built features that could be easily integrated with applications using APIs. The base CNN networks have provided many representations based on a large volume of datasets and learning processes in the past. Hence, in the transfer learning process, it is sufficient to merge the last few layers of the base CNN with the newer layers. In doing so, the bare minimal representations of figures and images, such as square shapes, outlines, curves, etc, can be utilized by the new algorithms that merge the transfer learning models.
Скачать Edge Intelligence: Deep Learning-enabled edge computing
