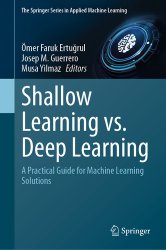
Автор: Ömer Faruk Ertuğrul, Josep M Guerrero, Musa Yilmaz
Издательство: Springer
Серия: The Springer Series in Applied Machine Learning
Год: 2024
Страниц: 283
Язык: английский
Формат: pdf (true), epub
Размер: 45.5 MB
This book explores the ongoing debate between shallow and Deep Learning in the field of Machine Learning. It provides a comprehensive survey of Machine Learning methods, from shallow learning to Deep Learning, and examines their applications across various domains. Shallow Learning vs Deep Learning: A Practical Guide for Machine Learning Solutions emphasizes that the choice of a Machine Learning approach should be informed by the specific characteristics of the dataset, the operational environment, and the unique requirements of each application, rather than being influenced by prevailing trends.
In each chapter, the book delves into different application areas, such as engineering, real-world scenarios, social applications, image processing, biomedical applications, anomaly detection, natural language processing (NLP), speech recognition, recommendation systems, autonomous systems, and smart grid applications. By comparing and contrasting the effectiveness of shallow and Deep Learning in these areas, the book provides a framework for thoughtful selection and application of Machine Learning strategies. This guide is designed for researchers, practitioners, and students who seek to deepen their understanding of when and how to apply different Machine Learning techniques effectively. Through comparative studies and detailed analyses, readers will gain valuable insights to make informed decisions in their respective fields.
- Compares and contrasts shallow learning and Deep Learning techniques, exploring their applications in various fields
- Emphasizes real-world applications of Machine Learning, exploring the strengths and limitations of the techniques
- Practical guide offers strategies for selecting the most suitable approach for researchers, practitioners, and students
As the field progressed, ML expanded to include more complex algorithms, such as Decision Trees, SVM, and kNN. These methods, which still fall under the umbrella of SL, represented a significant leap forward. They enabled machines to handle nonlinear relationships and more complex decision boundaries, thereby broadening the scope of ML applications. During this era, the focus was on enhancing the algorithms’ ability to generalize from limited data, a challenge that was central to the success of ML.
Скачать Shallow Learning vs. Deep Learning
