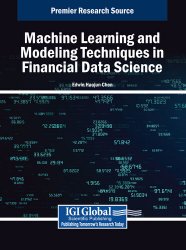
Автор: Haojun Chen
Издательство: IGI Global Scientific Publishing
Год: 2025
Страниц: 574
Язык: английский
Формат: pdf (true), epub
Размер: 34.1 MB
The integration of Machine Learning and modeling in finance is transforming how data is analyzed, enabling more accurate predictions, risk assessments, and strategic planning. These advanced techniques empower financial professionals to uncover hidden patterns, automate complex processes, and enhance decision-making in volatile markets. As industries increasingly rely on data-driven insights, the adoption of these tools contributes to greater efficiency, reduced uncertainty, and competitive advantage. This technological shift not only drives innovation within financial sectors but also supports broader economic stability and growth by improving forecasting and mitigating risks. Machine Learning and Modeling Techniques in Financial Data Science provides an updated review and highlights recent theoretical advances and breakthroughs in professional practices within financial data science, exploring the strategic roles of Machine Learning and modeling techniques across various domains in finance. It offers a comprehensive collection that brings together a wealth of knowledge and experience. Covering topics such as algorithmic trading, financial technology (FinTech), and natural language processing (NLP), this book is an excellent resource for business professionals, leaders, policymakers, researchers, academicians, and more.
One key area of research closely linked to algorithmic trading is financial market forecasting. Numerous studies examining the use of Machine Learning (ML) models, such as Random Forest and Support Vector Machines, alongside AI technologies like Artificial Neural Networks (ANN), have demonstrated their ability to predict the movements of global stock indices with impressive accuracy. More advanced AI techniques, including Long Short-Term Memory (LSTM) neural networks and ensemble methods such as XGBoost, have further enhanced predictive capabilities. These models consistently outperform market averages, challenging the weak form of the Efficient Market Hypothesis. The results suggest that AI has significant potential to exploit inefficiencies in capital markets, offering valuable tools for enhancing decision-making among investors and financial professionals. As AI technologies continue to evolve, future research will refine existing models and develop new ones, incorporating cutting-edge advancements in Machine Learning. Mastering the application of these technologies in financial markets has thus become a critical area of knowledge for financial professionals.
In addition to predictive algorithms, another powerful AI tool gaining traction in finance is Natural Language Processing (NLP). While traditional financial models focus on numeric data, NLP taps into vast amounts of unstructured text data, such as news articles, earnings reports, and social media content, to extract valuable insights into market sentiment and trends. As NLP continues to evolve, its integration into financial decision-making is becoming increasingly essential, particularly in areas such as credit risk analysis, investor sentiment studies, and financial auditing. NLP enables financial professionals to make more informed, timely decisions based not only on historical financial metrics but also on real-time market sentiment and external factors. However, as this technology advances, challenges emerge, especially in terms of the accuracy of sentiment analysis and the risk of information overload. For instance, automated sentiment analysis models must be carefully calibrated to avoid misinterpreting nuanced sentiment, ensuring that insights are both reliable and actionable. While the ability to process vast volumes of textual information quickly can revolutionize financial analysis, it also raises concerns about ensuring that the results remain grounded in verifiable, meaningful data.
As financial markets become more data-driven and the influence of AI deepens, emerging technologies like Quantum Machine Learning (QML) are expected to play an increasingly significant role. Quantum computing, which uses quantum bits (qubits) capable of representing multiple states simultaneously, promises a leap in computational power, enabling more sophisticated modeling and optimization in financial applications. This breakthrough could revolutionize processes such as portfolio optimization and real-time market simulations. While quantum computing is still in its early stages, its integration with machine learning techniques offers the potential to accelerate financial decision-making to unprecedented levels, heralding the dawn of a new era in financial technology.
Скачать Machine Learning and Modeling Techniques in Financial Data Science
