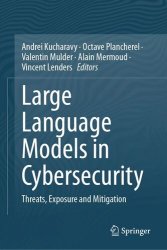
Автор: Andrei Kucharavy, Octave Plancherel, Valentin Mulder, Alain Mermoud
Издательство: Springer
Год: 2024
Страниц: 249
Язык: английский
Формат: pdf (true)
Размер: 11.3 MB
This book provides cybersecurity practitioners with the knowledge needed to understand the risks of the increased availability of powerful large language models (LLMs) and how they can be mitigated. It attempts to outrun the malicious attackers by anticipating what they could do. It also alerts LLM developers to understand their work's risks for cybersecurity and provides them with tools to mitigate those risks. The book starts in Part I with a general introduction to LLMs and their main application areas. Part II collects a description of the most salient threats LLMs represent in cybersecurity, be they as tools for cybercriminals or as novel attack surfaces if integrated into existing software. Part III focuses on attempting to forecast the exposure and the development of technologies and science underpinning LLMs, as well as macro levers available to regulators to further cybersecurity in the age of LLMs. Eventually, in Part IV, mitigation techniques that should allow safe and secure development and deployment of LLMs are presented. The book concludes with two final chapters in Part V, one speculating what a secure design and integration of LLMs from first principles would look like and the other presenting a summary of the duality of LLMs in cyber-security. While the general public discovered Large Language Models (LLMs) with ChatGPT—a generative autoregressive model, they are far from the only models in the LLM family. Various architectures and training regiments optimized for specific usages were designed throughout their development, which were then classified as different LLM families.