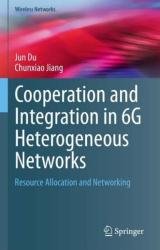
Автор: Jun Du, Chunxiao Jiang
Издательство: Springer
Серия: Wireless Networks
Год: 2023
Страниц: 461
Язык: английский
Формат: pdf (true)
Размер: 14.3 MB
The first book on the multi-dimensional resource management for heterogenous communication, computing and caching in 6G.
To provide ubiquitous and various services, 6G networks tend to be more comprehensive and multidimensional by integrating current terrestrial networks with space-/air-based information networks and marine information networks; then, heterogeneous network resources, as well as different types of users and data, will be also integrated. Driven by the exponentially growing demands of multimedia data traffic and computation-heavy applications, 6G heterogenous networks are expected to achieve a high QoS with ultra-reliability and low latency. In response, resource allocation has been considered an important factor that can improve 6G performance directly by configuring heterogeneous communication, computing and caching resources effectively and efficiently.
The book addresses a range of technical issues in cooperative resource allocation and information sharing for the future 6G heterogenous networks, from the terrestrial ultra-dense networks and space-based networks to the integrated satellite-terrestrial networks, as well as introducing the effects of cooperative behavior among mobile users on increasing capacity, trustworthiness and privacy. For the cooperative transmission in heterogeneous networks, the authors commence with the traffic offloading problems in terrestrial ultra-dense networks, and the cognitive and cooperative mechanisms in heterogeneous space-based networks, the stability analysis of which is also provided.
Moreover, for the cooperative transmission in integrated satellite-terrestrial networks, the authors present a pair of dynamic and adaptive resource allocation strategies for traffic offloading, cooperative beamforming and traffic prediction based cooperative transmission. Later, the authors discuss the cooperative computation and caching resource allocation in heterogeneous networks, with the highlight of providing our current studies on the game theory, auction theory and deep reinforcement learning based approaches. Meanwhile, the authors introduce the cooperative resource and information sharing among users, in which capacity oriented-, trustworthiness oriented-, and privacy oriented cooperative mechanisms are investigated. Finally, the conclusion is drawn.
Recently, the fifth-generation (5G) of wireless network is developed to support enhanced mobile broadband (eMBB), massive machine-type communications (mMTC) and ultra-reliable and low-latency communications (uRLLC), according to the report of International Telecommunication Union (ITU). Benefitting from such high performance, 5G has opened new doors of opportunity towards emerging applications, e.g., augmented reality (AR), virtual reality (VR), tactile reality, mixed reality, etc. However, the new media, such as holographic communications, will request much higher transmission speeds up to Tera bits per second (Tbit/s) than AR and VR. Then 5G is far from enough to support the faster, more reliable and larger scale communication requirements of these services. In response, the investigation of future generation wireless networks (6G) has been triggered, which promises more powerful capacities in terms of ultra-broadband, supper massive access, ultra-reliability and low-latency than 5G does.
In recent years, AI is emerging as a fundamental paradigm to orchestrate the communication and information systems from bottom to top. For the foreseeable future, AI-enabled networks will open up new opportunities for the smart and intelligent 6G networking. As a major branch of AI, ML can establish an intelligent system which operates in the complicated environment. Recently, ML has mainly developed into many branches such as classical ML, including supervised learning and unsupervised learning, Deep Learning (DL) and reinforcement learning (RL). DL aims to understand the representations of data, and can be modeled in supervised, unsupervised, and reinforcement learning. Different AI/ML techniques, such as RL, DRL, Double DRL (DDRL), etc., have been introduced to cooperative networking and resource allocation techniques, in order to deal with the sophisticated optimization of decision making resulting from the multi-dimension, randomly uncertainty and dynamics of 6G. By applying AI/ML tools, the valuable information can be extracted through training observed data, and then different functions for prediction, optimization, and decision making in traffic offloading, caching and cloud/fog/edge computing can be learned to support ultra-reliable and low-latency services.
Скачать Cooperation and Integration in 6G Heterogeneous Networks: Resource Allocation and Networking
