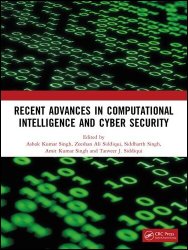
Автор: Ashok Kumar Singh, Zeeshan Ali Siddiqui, Siddharth Singh, Amit Kumar Singh
Издательство: CRC Press
Год: 2024
Страниц: 400
Язык: английский
Формат: pdf (true)
Размер: 32.8 MB
In the ever-accelerating tapestry of our digital age, the symbiotic relationship between computational intelligence and cyber security has become the linchpin of progress. The relentless pace of technological evolution and the ceaseless emergence of cyber threats demand not only adaptation but also an exploration of the forefronts of innovation and defence. Recent Advances in Computational Intelligence and Cyber security is a testament to the exhilarating journey undertaken by researchers, practitioners, and visionaries in these pivotal fields. Within the confines of this book, we embark on a captivating exploration of the cutting-edge developments that define the current state of computational intelligence and the intricate dance with the ever-evolving landscape of cyber security.
Creating models and methods that will enable computer systems to replicate human intellect is the aim of the Computer Science field of Artificial Intelligence (AI). That’s why cybersecurity is getting more and more crucial. Preventing data breaches, financial losses, and privacy violations requires effective network security and information security. This clarifes the growing signifcance of cybersecurity. Preventing financial losses, privacy violations. Instantaneous analysis and prioritization of alarms are possible with AI-powered systems, enabling quick decision-making and proactive threat containment. AI improves the effectiveness of security teams by automating repetitive operations, allowing them to devote more time to strategic threat identifcation and vulnerability analysis. AI-generated real-time threat intelligence enables enterprises to anticipate possible weaknesses and proactively strengthen their defenses, providing a strong security posture. However, the symbiotic relationship between AI and cybersecurity is with challenges. Adversaries may be able to leverage AI algorithms for their own advantage or launch attacks that bypass AI-based defenses, demanding a perpetual cycle of innovation and protection. Furthermore, regulation and comprehensive research of the ethical concerns surrounding AI’s participation in autonomous decision-making and privacy violations are required. Finally, the importance of AI in cybersecurity cannot be overstated.
In this chapter we have presented a working model for the detection of the genuine and fake Domain Name System (DNS) traffc in internet service providers (ISP) network traffic using Data Science and Machine Learning (ML). When any host is accessing the network for DNS query or mail exchange records. Based on the source port, destination port, source address and destination address we recorded the net-fow traffic from the router port using the various port mirroring techniques for a certain time period. A pattern was used with the number of DNS requests and their response for each record. Two ML patterns were used K-nearest neighbors (KNN), Naive Bayes (NB) for classifcation purposes and using it further to predict and analyze the behavior in the data. We used the prediction to classify the accuracy and training time as metrics of performance to find out which algorithm is more accurate than the other. The data set used in training and testing the algorithms contains several types of malicious as well as genuine ports for classifcation.
Optimizing hyperparameters in neural networks is crucial for achieving peak performance. Traditional methods involve time-consuming trial and error, making it challenging to find the best hyperparameter settings. In this research, we introduce Genetic Algorithms (GAs) as an efficient approach for hyperparameter optimization. Using a real-world diabetes diagnosis dataset, we cast hyperparameter tuning as an optimization problem. We create a fitness function to assess neural network models based on essential hyperparameters, such as learning rate, beta1, beta2, and epsilon. The GA evolves a population of candidate solutions over generations, effciently exploring the hyperparameter space. Results show that the proposed approach consistently converges to hyper-parameter values enhancing model accuracy and training convergence. We analyze the convergence curve, fitness value distribution, and hyperparameter evolution. This study reveals GAs as a potent automated tool for neural network hyperparameter tuning. It saves time and computational resources, advancing model development. Insights gained improve understanding of hyperparameter impacts on neural network performance.
Скачать Recent Advances in Computational Intelligence and Cyber Security
