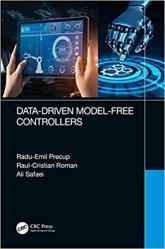
Автор: Radu-Emil Precup, Raul-Cristian Roman
Издательство: CRC Press
Год: 2022
Страниц: 403
Язык: английский
Формат: pdf (true)
Размер: 19.2 MB
This book categorizes the wide area of data-driven model-free controllers, reveals the exact benefits of such controllers, gives the in-depth theory and mathematical proofs behind them, and finally discusses their applications. Each chapter includes a section for presenting the theory and mathematical definitions of one of the above mentioned algorithms. The second section of each chapter is dedicated to the examples and applications of the corresponding control algorithms in practical engineering problems. This book proposes to avoid complex mathematical equations, being generic as it includes several types of data-driven model-free controllers, such as Iterative Feedback Tuning controllers, Model-Free Controllers (intelligent PID controllers), Model-Free Adaptive Controllers, model-free sliding mode controllers, hybrid model‐free and model‐free adaptive‐Virtual Reference Feedback Tuning controllers, hybrid model-free and model-free adaptive fuzzy controllers and cooperative model-free controllers.
Reinforcement Learning (RL) is a data-driven and also ML technique whose specific feature is the use of information gathered from interactions with the environment. The RL problem is formulated in the Markov decision process framework using dynamic programming to solve the optimization problem that ensures optimal reference tracking. An RL agent executes actions in the environment and based on the received reward, adjusts its knowledge about itself and the environment. By applying this process incrementally, the RL agent will become better at picking actions, which will maximize or minimize the rewards. RL is a viable technique that solves optimal reference tracking problems and fills the gap between ML and control. In this context, the RL agent is the controller that automatically learns how to modify its parameters and how to control a process based on the feedback (reward) it receives from it.
The book includes the topic of optimal model-free controllers, as well. The optimal tuning of model-free controllers is treated in the chapters that deal with Iterative Feedback Tuning and Virtual Reference Feedback Tuning. Moreover, the extension of some model-free control algorithms to the consensus and formation-tracking problem of multi-agent dynamic systems is provided. This book can be considered as a textbook for undergraduate and postgraduate students, as well as a professional reference for industrial and academic researchers, attracting the readers from both industry and academia.
Table of Contents:
1. Introduction. 2. Iterative Feedback Tuning. 3. Intelligent PID Controllers. 4. Model-Free Sliding Mode Controllers. 5. Model-Free Adaptive Controllers. 6. Hybrid Model-Free and Model-Free Adaptive Virtual Reference Feedback Tuning controllers. 7. Hybrid model-free and model-free adaptive fuzzy controllers. 8. Cooperative Model-Free Adaptive Controllers for Multi-Agent Systems. Appendix 1. Simulation resutls for implementation of Model-Free Adaptive Controller on a differential-drive ground mobile robot.
Скачать Data-Driven Model-Free Controllers
