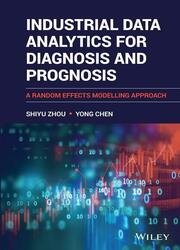
Автор: Shiyu Zhou, Yong Chen
Издательство: Wiley
Год: 2021
Страниц: 353
Язык: английский
Формат: pdf (true)
Размер: 12.95 MB
Discover data analytics methodologies for the diagnosis and prognosis of industrial systems under a unified random effects model.
In Industrial Data Analytics for Diagnosis and Prognosis - A Random Effects Modelling Approach, distinguished engineers Shiyu Zhou and Yong Chen deliver a rigorous and practical introduction to the random effects modeling approach for industrial system diagnosis and prognosis. In the book’s two parts, general statistical concepts and useful theory are described and explained, as are industrial diagnosis and prognosis methods. The accomplished authors describe and model fixed effects, random effects, and variation in univariate and multivariate datasets and cover the application of the random effects approach to diagnosis of variation sources in industrial processes. They offer a detailed performance comparison of different diagnosis methods before moving on to the application of the random effects approach to failure prognosis in industrial processes and systems.
In addition to presenting the joint prognosis model, which integrates the survival regression model with the mixed effects regression model, the book also offers readers:
A thorough introduction to describing variation of industrial data, including univariate and multivariate random variables and probability distributions
Rigorous treatments of the diagnosis of variation sources using PCA pattern matching and the random effects model
An exploration of extended mixed effects model, including mixture prior and Kalman filtering approach, for real time prognosis
A detailed presentation of Gaussian process model as a flexible approach for the prediction of temporal degradation signals
Ideal for senior year undergraduate students and postgraduate students in industrial, manufacturing, mechanical, and electrical engineering, Industrial Data Analytics for Diagnosis and Prognosis is also an indispensable guide for researchers and engineers interested in data analytics methods for system diagnosis and prognosis.
Скачать Industrial Data Analytics for Diagnosis and Prognosis
