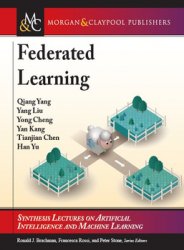
Автор: Qiang Yang, Yang Liu
Издательство: Morgan & Claypool
Год: 2019
Страниц: 209
Язык: английский
Формат: pdf (true)
Размер: 10.1 MB
How is it possible to allow multiple data owners to collaboratively train and use a shared prediction model while keeping all the local training data private? Traditional Machine Learning approaches need to combine all data at one location, typically a data center, which may very well violate the laws on user privacy and data confidentiality. Today, many parts of the world demand that technology companies treat user data carefully according to user-privacy laws. The European Union's General Data Protection Regulation (GDPR) is a prime example. In this book, we describe how Federated Machine Learning addresses this problem with novel solutions combining distributed machine learning, cryptography and security, and incentive mechanism design based on economic principles and game theory. We explain different types of privacy-preserving machine learning solutions and their technological backgrounds, and highlight some representative practical use cases. We show how federated learning can become the foundation of next-generation machine learning that caters to technological and societal needs for responsible AI development and application.
This book is about how to build and use machine learning (ML) models in artificial intelligence (AI) applications when the data are scattered across different sites, owned by different individuals or organizations, and there is no easy solution to bring the data together. Nowadays, we often hear that we are in the era of big data, and big data is an important ingredient that fuels AI advances in today’s society. However, the truth is that we are in an era of small, isolated, and fragmented data silos. Data are collected and located at edge devices such as mobile phones. Organizations often have limited views on users’ data due to their specialties. However, privacy and security requirements make it increasingly infeasible to merge the data at different organizations in a simple way. In such a context, federated machine learning (or federated learning, in short) emerges as a functional solution that can help build high-performance models shared among multiple parties while still complying with requirements for user privacy and data confidentiality.
Скачать Federated Learning (Synthesis Lectures on Artificial Intelligence and Machine Learning)
