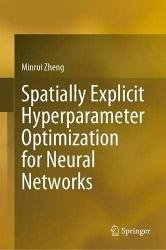
Автор: Minrui Zheng
Издательство: Springer
Год: 2021
Страниц: 120
Язык: английский
Формат: pdf (true), epub
Размер: 36.4 MB
Neural networks as the commonly used machine learning algorithms, such as artificial neural networks (ANNs) and convolutional neural networks (CNNs), have been extensively used in the GIScience domain to explore the nonlinear and complex geographic phenomena. However, there are a few studies that investigate the parameter settings of neural networks in GIScience. Moreover, the model performance of neural networks often depends on the parameter setting for a given dataset.
Meanwhile, adjusting the parameter configuration of neural networks will increase the overall running time. Therefore, an automated approach is necessary for addressing these limitations in current studies. This book proposes an automated spatially explicit hyperparameter optimization approach to identify optimal or near-optimal parameter settings for neural networks in the GIScience field. Also, the approach improves the computing performance at both model and computing levels. This book is written for researchers of the GIScience field as well as social science subjects.
Artificial neural networks (ANNs) are an inductive machine learning approach that resembles the brain functions of human beings or animals for problem-solving. ANNs are one of the powerful approaches in scientific and engineering applications when used to predict or recognize patterns. ANNs can learn from data to improve their performance and adapt themselves as more data are available. ANNs offer an alternative way of constructing complex models by allowing for representing nonlinear relationships via directly learning from data. There are different types of ANNs regarding the network architecture: for example, feed-forward neural network, radial basis function network, and recurrent networks. Although various types of neural network models have been developed, the one that is most widely used is the feed-forward neural network. The training of a neural network is to use learning algorithms (i.e. supervised learning algorithm) to adjust these weights to minimize the error in outputs, thus determining the optimal network setting for problem-solving.
