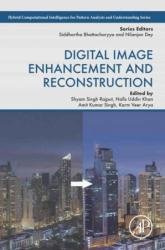
Автор: Shyam Singh Rajput, Nafis Uddin Khan, Amit Kumar Singh
Издательство: Academic Press/Elsevier
Серия: Hybrid Computational Intelligence for Pattern Analysis and Understanding
Год: 2023
Страниц: 369
Язык: английский
Формат: pdf
Размер: 18.7 MB
Digital Image Enhancement and Reconstruction: Techniques and Applications explores different concepts and techniques used for the enhancement as well as reconstruction of low-quality images. Most real-life applications require good quality images to gain maximum performance, however, the quality of the images captured in real-world scenarios is often very unsatisfactory. Most commonly, images are noisy, blurry, hazy, tiny, and hence need to pass through image enhancement and/or reconstruction algorithms before they can be processed by image analysis applications. This book comprehensively explores application-specific enhancement and reconstruction techniques including satellite image enhancement, face hallucination, low-resolution face recognition, medical image enhancement and reconstruction, reconstruction of underwater images, text image enhancement, biometrics, etc. Chapters will present a detailed discussion of the challenges faced in handling each particular kind of image, analysis of the best available solutions, and an exploration of applications and future directions. The book provides readers with a deep dive into denoising, dehazing, super-resolution, and use of soft computing across a range of engineering applications.
Since the recent decades there have been increasing high-speed technological innovations, such as automatic industrial production, intelligent lifestyles, and digital information transmission and acquisition, with which original paper books and newspapers being gradually replaced by video images. For example, video images are widely used in a criminal inquiry, vehicle tracking, flow monitoring, and medical diagnosis. Under such conditions, the video image quality may directly determine relevant data and information acquisition effect, and, sometimes, low-quality images may cause property losses or threaten social and public security. Therefore ever-more focus has been put on the low-quality video Image Enhancement (IE) through Artificial Intelligence (AI) algorithms.
Deep Learning (DL) is one of the AI algorithms. The Convolutional Neural Network (CNN) has shown great potential in image recognition and has made many attempts in the field of IE of underlying vision. For example, the CNN can convert the IE problems into the regression from the degraded low-quality image to the original high-quality clear image, and the End-To-End (ETE) network training is realized through the sample pairs of low-quality image and high-quality image to learn and obtain this regression mapping. However, there are two problems in the application of CNN to IE: firstly, CNN uses its powerful nonlinear representation ability to approximate clear images at the pixel level and does not learn the manifold distribution of the original high-quality clear images; secondly, most CNN learn more image features by designing deeper or wider networks to improve its representation ability, but they do not make full use of the inherent interdependence of feature channels. Generic Adversarial Networks (GAN) can force the distribution of the reconstructed image to approach the real image manifold distribution by adding additional adversarial networks, thus producing a reconstructed image with a better visual effect. The channel Attention Mechanism (AM) can give different weights to the feature channels so that the network can selectively emphasize the features conducive to IE and suppress the less useful features. Finally, high-quality and clear real images, the same as the real image data, are generated, meeting the needs of data enhancement. Therefore using GAN to complete effective image data enhancement has important research significance.
Скачать Digital Image Enhancement and Reconstruction (Hybrid Computational Intelligence for Pattern Analysis and Understanding)
